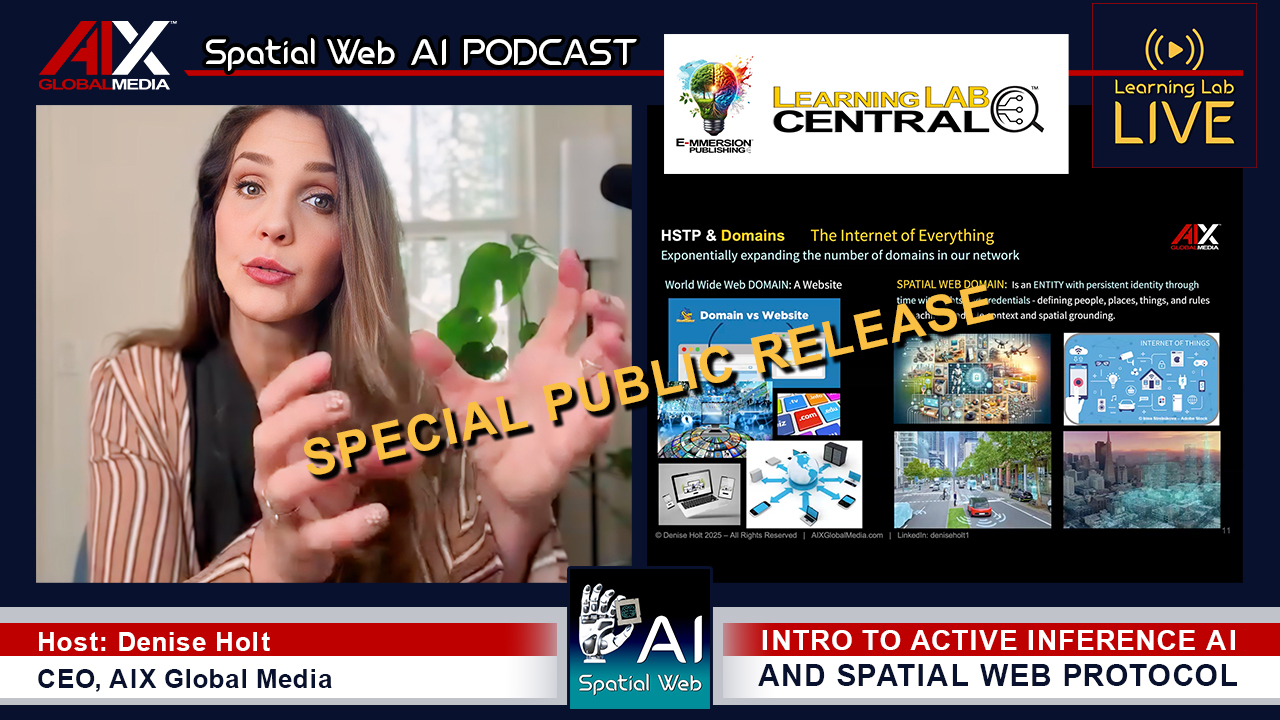
The convergence of two groundbreaking technologies is reshaping how we think about AI, automation, and intelligent systems, affecting every industry...
The collective progress in this field showcases Active Inference’s potential as a systematic blueprint for Artificial General Intelligence (AGI).”
As Chief Scientist at VERSES AI and pioneer of the Free Energy Principle that underlies Active Inference, Friston and his VERSES team have developed an entirely new kind of AI that mimics biological intelligence, able to take any amount of data and make it ‘smart’.
In an exclusive presentation at the recent 4th International Workshop on Active Inference (IWAI) held in Ghent, Belgium this past September 13–15, 2023, world-renowned neuroscientist Dr. Karl Friston gave fewer than 100 global Active Inference experts and researchers a glimpse into the future of next generation artificial intelligence. His talk provided perspective into some of the latest research related to state spaces, universal generative models, belief sharing between agents, active learning, and structured learning.
Dr. Friston is at the forefront of developing ‘Active Inference’ models, revolutionizing how intelligent systems learn and adapt. The information shared at IWAI is built on more than a decade’s groundbreaking work and collaboration with fellow researchers in the Nested Minds Network at University College London.
At the heart of understanding intelligence lies the idea of ‘generative world models’ — these are probabilistic frameworks that encapsulate the underlying structure and dynamics involved in the creation of sensory data. As Friston explained, developing a universal generative world model that can be widely applied has been a core pursuit.
The challenge lies in modeling the intricacies of ‘state spaces’ — capturing not just sensory data itself but the hidden variables and control factors that determine how stimuli unfold over time. By incorporating hierarchical and factorial structure, increased temporal depth, and an emphasis on Agent dynamics and control paths, Friston has worked to construct expressive yet interpretable models of complex environments.
As he suggested, the resulting grammar of cognitive machinery — factor graphs equipped with message passing — may provide a foundation for advancing distributed and embedded intelligence systems alike.
A significant portion of the talk focused on state spaces within these generative models. Friston highlighted how understanding these spaces is vital for grasping the dynamics of how our brain predicts and reacts to stimuli.
Friston has focused heavily on developing more complete generative models of disputed state spaces, generalizing and expanding on partially observed Markov decision processes. His models place special emphasis on representing paths and control variables over time, hierarchical framework through various time scales, while accounting for a variety of factors that can operate independently or in conjunction with each other, across different layers or levels of the model. The direct modeling of dynamics and control in his work sheds new light on understanding concepts like intentional planning as opposed to behaviors influenced by prior preferences.
Intelligence also relies on interacting with other Agents. Friston showed that when Intelligent Agents pursue ‘active learning’ with a focus on gaining information, this approach inherently leads to the development of common communication methods and linguistic structures. Essentially, as Agents strive to enhance their model’s accuracy and effectiveness by exchanging beliefs, self-organized patterns evolve, spontaneously establishing a basis for mutual understanding and predictability.
Developing methods for seamlessly pooling knowledge and achieving consensus, while retaining specialization, is an active research direction. The integrated components must balance stability with flexibility, adapting core beliefs slowly while remaining receptive to external influences. As Friston noted, much like human maturational trajectories, childlike malleability fades, yet the integration of new ideas remains possible through through niche environments.
A universal ‘world model’ that can be widely applied remains an ambitious goal. Friston discussed two potential approaches — an initially simple model that grows structurally to capture more complexity versus a massively interconnected model that relies more on attentional spotlights. He also noted the potential for composing such models from subgraphs of specialized Intelligent Agents, allowing for parallel development of appropriately-scoped pieces. Belief sharing through communication protocols, such as the Spatial Web Protocol developed by VERSES AI, enables the learning Agents to converge on a mutually predictable, and thereby more efficient, joint world model.
Belief sharing and its impact on active learning formed another cornerstone of Dr. Friston’s presentation. He revealed how our brains share and update beliefs, a process central to learning and adapting to new environments.
In his talk, Friston demonstrated belief sharing in an example with multiple Intelligent Agents playing a hiding game. By actively learning the mapping between beliefs and communicative utterances, the Agents can resolve uncertainty and synchronize their internal models via the utterances that propagate beliefs. This emergent communication system arises naturally from the drive to maximize the information and efficiency of the shared world model.
In a more technical sense, Friston views active learning as a process where updates to the model are seen as actions aimed at reducing expected free energy. This perspective is rooted in information theory, with free energy representing the gain in information, bounded by certain preferences, which arises from updating specific parameters. As a result, the model learns mappings that are both sparse and rich in mutual information, effectively representing structured relationships. Friston illustrated this through a simulation of Intelligent Agents jointly self-organizing around a shared language model.
Finally, structured learning was discussed as a novel approach within the realm of active inference. Dr. Friston provided insights into how structured learning frameworks could offer more efficient ways of understanding and interacting with the world.
Friston explored active model selection, framing the expansion of a model’s structure as similar goal-directed optimization through expected free energy. Gradually introducing new elements such as states or factors, following simple heuristic principles, allows for the automatic expansion of the model’s complexity, to better reflect and improve its alignment with the learning environment. Friston demonstrated applications ranging from unsupervised clustering to the development of distinct, non-overlapping representations in an Agent simulation.
Intelligence requires not just statistical modeling, like what we see in current AI machine learning/deep learning systems, but recognizing structural composition — the patterns of parts to wholes and dependencies that characterize natural systems that occur in the real world. Friston outlined strategies for progressively evolving the architecture of real-world models, making improvements to modular elements in response to fresh data, all centered around clear goals.
The guided increase in complexity astonishingly leads to the natural development of organized and distinct feature areas, classifies them into clear categories, and controls their behavior patterns, all from raw multimedia data streams. Such capabilities get closer to flexible human-like learning that moves far beyond the current passive pattern recognition systems of today, into Autonomous Intelligent Systems interacting with and reshaping their environments.
Overall, Dr. Karl Friston’s presentation at IWAI 2023 demonstrates that his work continues to push Active Inference AI into new territory through innovations like inductive planning, information-theoretic formalisms, emergent communication systems, and structured learning. As the models, methods, and insights mature, the prospects grow ever stronger for realizing artificial intelligence as multitudes of Intelligent Agents that can learn, act, and cooperate at the level of human intelligence.
While Friston’s presentation was meant only as a glimpse, these insights keep VERSES AI at the leading edge of creating Autonomous Intelligent Systems (AIS) that can understand, communicate, and adapt as humans do. The foundation of Dr. Karl Friston’s impact in the realm of AI is driven by his clarity of vision and deep intellectual insight that are actively shaping the evolution of this next era of these technologies.
00:00 — Introduction by Tim and Karl Friston’s Opening Remarks
06:09 — The Significance of Free Energy in Understanding Life
07:10 — Exploring Markov Blankets and Factor Graphs
09:56 — Hierarchical Generative Models and Time Perception
13:36 — Active Inference and Planning with Expected Free Energy
15:40 — Joint Free Energy and Generalized Synchrony in Physics
16:40 — The Role of Paths and Intentions in Physics and Active Inference
17:35 — Specifying Endpoints of Paths as Goals
30:26 — Communication and Belief Sharing in Federated Systems
31:35 — Generative Models and Perception in Agents
40:19 — Model Comparison and Latent State Discovery
41:25 — Active Model Selection and Handwritten Digit Styles
45:30 — Structured Learning and Object Localization
49:10 — Epistemic Foraging and Confidence in Learning
50:21 — The Dynamics of Information Gain and Learning
51:29 — Conclusion and Q&A Begins
01:19:33 — Emphasis on Constraints in the Free Energy Principle
01:23:40 — Closing Remarks
01:24:55 — Karl Friston’s Reflections on the Field’s Evolution and the Next Generation
All content for Spatial Web AI is independently created by me, Denise Holt.
Empower me to continue producing the content you love, as we expand our shared knowledge together. Become part of this movement, and join my Substack Community for early and behind the scenes access to the most cutting edge AI news and information.
00:00
We’re here at the international workshop of Active Inference in Ghent, Belgium. I’m a PhD student in cognitive computing. It.
01:15
So I’m more than happy that this person doesn’t need any introductions for you. So I’ll just give it up for.
01:27
Thank you very much. I can see you there. It’s a great pleasure. It’s a great pleasure to be able to. I’ve really enjoyed the conference so far. My job, usually at this stage, is to try and summarize the cross cutting themes and celebrate the diversity of the things that we’ve been enjoying over the past couple of days. But Tim asked me to be more self indulgent and reflect upon things that me and my colleagues have been focusing on in the past year. So what I want to do is just briefly rehearse some of the things that we’ve been focusing on, and I’m going to try to pick up on some of the questions and the intriguing issues that you’ve encountered over the past couple of days as I go.
02:24
But there were so many of them, I’m going to forget, I’m sure most of them. So I’ve noticed that Tim has given me an hour and a half, which seems a little bit luxurious. So what I imagine will happen is I’ll talk for, I would hope, about 40 plus minutes, answer questions, and then at Tim’s discretion, then segue into a more general discussion, an open discussion of the emerging themes, the retrospective on the workshop itself. So I said, it’s self indulgent. It’s not that self indulgent. What I’m going to be talking about are themes and ambitions that have been worked up with colleagues from a number of spheres. And in fact, most of my colleagues actually sitting in the audience, primarily from UCL versus with interest from the active influence institute.
03:26
And some of this work has been motivated by a commitment to contributing to IGP standards for the next kind of message passing protocols on the web, as represented by the space the Web foundation. So I identified five themes. The most generic theme is trying to develop an expressive and universal generative model for dispute state spaces. That model can be thought of as a generalization or a more complete kind of partial Markov decision process, with a special emphasis on the rule of paths and how paths may or may not be controllable, the hierarchical structure and what that means in terms of separation of temporal scales and how that speaks to what Bert was talking about in terms of reactive message passing. The specifics of what this problem brings to the table.
04:32
I’m going to cover in terms of development active inference, specifically what is afforded by having an explicit representation of paths and the notion of inductive influence, or putting inductive influence into active influence. Talking about generalizations of active learning in the sense that active learning can be equipped with a very special meaning in context of active influence. If we look at learning the updating of model parameters as an action, and if it is an action, then it succumbs to the same principles as any other action, namely to maximize the prospective reality of actions pertaining to the parameters. And we’ll see what that means. You already know what it means because you’ve been talking about it, but it’s nice to unpack it and relate it to established ideas and illustrate active learning in the context of federated influence and belief sharing, and then finally active selection.
05:32
Again the same notion of thinking about structure learning basic model comparison as an active presence of comparing moral model with another model, and again leveraging the notion that every action, every move we make, including the way that we update our models, can be expressed as a process of minimizing expected free energy. And I’ll illustrate that in the context of disentanglement. So that’s the idea. Start at the beginning. The nature of things and how they behave. I’ve just summarized that here. Can you maybe go to presentation mode?
06:18
The free energy principle as we’ve seen many times with different guises throughout the workshop, I’m just summarizing action and perception as the minimization of free energy with respect to action, and the optimization of the internal states representing external states minimizing a generalized free energy that subsumed expected free energy that has the normal interpretations in terms of expected information gain and expected utility or prior preferences. So that’s the idea that introduces the notion. One of the tenets of his account is that living systems remain organized because they feed upon negative entropy. And what I’d repeat, I’d forgotten or didn’t know, is that in the second edition of what is life strobe states, if I had been catering for them merely physicists alone, I should have let the discussion return on free energy. Instead.
07:19
It is the more familiar notion in this context that this seems behind a technical term, or this highly technical term seems linguistically too near to energy for making the average reader alive to the contrast between the two things. So it’s a real shame that he didn’t use free energy, because that would have made certainly our lives easier, possibly Richard fines. So back to the Markov blanket, just to demystify that for our friends from outside the activating institute, it’s just a specification of the ports or the inputs and outputs to a system where we are focusing on the internal organisation that mediates the inputs and the outputs of the action perception cycle. And as we have come from men perspectives, this can be characterized as message passing on a factor graph. And that’s a theme that I’m going to develop.
08:19
But of course for every factor graph there is a graphical geriatric model and vice versa. So what kind of model have we been looking at from the past year? Effectively we’ve been looking at a generalization of Markov decision processes that foreground the role of dynamics or control variables or generally paths, so that the transition dynamics depend upon a particular path. But of course in the context of active inference and control, the paths themselves have a dynamics and of course the path, the next control variable, could either depend upon the previous control variable, say if I couldn’t control it, or if I can control it, say I have some prior preferences and underwriting expected free energy, then the path will change to the path of least action or the path of the expected free energy, denoted by g here from each given path k.
09:26
So c takes on a new role here as dynamics on things that are controllable, things that I can force in terms of changing the dynamics on the velocity encoded by b. And then we have the normal duration of parameterization into speed state space models leading to this graphical model here that has a temporal depth, simply because we’re paying careful attention to the dynamics and the states, and in this instance the dynamics among over facts here with their initial conditions and the usual likelihood mapping. To make it completely general, of course we need to equip it with higher graphical depth. This is interesting because it brings to the table the notion that a state at one level basically entails a trajectory, a path or a narrative at the lower level, say a spatial temporal receptive field, for example.
10:21
And finally, of course, we’ve also heard about the importance of mean field approximation and factorization within any level, at all levels of a hierarchical generative model, both in terms of the paths and in terms of the hidden state. So we have three characteristic kinds of depth to these deep generative models, temporal, hierarchical and factorial. And that hierarchical structure has, I think an interesting interpretation in relation to the temporal depth, because by construction it’s difficult to imagine how else you would construct it. What one is inducing in this hierarchical structure is a sense of pressure, of temporal scales, in the sense a state at one level maps to a path that has some universal clock at time associated with it at the lower level.
11:22
Then an instant at the higher level corresponds to a chunk of time at the lower level, a sequence of discrete events at the lower level, which necessarily means that the deeper I move into my hierarchical generative model, the more I progress towards the center. Things slow down, and I’ve tried to cartoon that here. In terms of the updates over time that will proceed relatively slowly, or you encounter less changes as you get deeper into the model and more changes at the extremities or the periphery of the model, the external Markoff blanket, as it were. So that’s, I think, a useful theme, which I’ll just briefly recapitulate in a couple of moments. But let’s just look at the corresponding factor graph. Magnus is going to be very crossover, because this is a noble slide that does not conform to the normal standards.
12:20
I’m sure it will. Forgive me. So here’s the equivalent factor graph with the random variable for states in the past on the edges, and the factors of the conditional probability distributions associated with these various tenses parameterized by judicial parameters here. And then, given this derivative model, it is a simple matter just to write the update rules. There’s no wiggle room here. They all fall out of the kind of universal or message passing scheme that Bernie was talking about. That is, in fact, just based upon variation free energy, giving you, in some instances, a mixture of belief propagation and variational message passing. I won’t bother going through them. But other than to note they rest upon a very small number of operators, maybe from a computer science point of view, basically tensor operators, some product operators, and a couple of nonlinearities.
13:25
Matter of fact, I just listed all the nonlinearities you would need here. Just a handful of nonlinearities, nonlinear morphisms and tensor some product operators, and that’s it. This should, in principle, be applicable to any given geographic model. You had in mind, indispute states, and would thereby allow you to build geography models if we have the right kind of software package, and we are moving towards those with things like Rxinfa and constpy MDP. One interesting twist here is to think about where is agency and where are agents? And is this hierarchical genetic model a composition of different genitive models in one big genitive model? Agency in this context inherits from father in the sense that if an agent has agency, she has to imagine the consequences of her actions.
14:28
That is slightly distinct, I think, from control as inference that we heard about in the last presentation, which would go a long way. But in active infants, we’re moving to planning as infants with an exquisite model of the future, where we score the quality of our plans in terms of the expected free energy. And we know the form of that from the patent formulation, from the free energy principle, because we know the form, we know the dependencies or the messages that we need from other parts of the graph in order to constitute the expected free energy that underlies the selection of particular plans and implicit agency. And interestingly, it falls down to ABC.
15:11
So I’m just making a sort of conceptual point, which may not be pragmatically useful, that an agent just is a collection of a, b’s and c’s, but it can be embedded in a much deeper, hierarchically structured graph. And I’ve illustrated the kind of graphs that we have in mind that may be a useful metaphor that says transactions message passing on the graph, where the graph is now conceived of, I would say a web or Internet web three.
15:48
So here’s our little subgraph here with the agents here, and I’ve just squashed it here and then arranged it in this deep hierarchical form, just to give a visual impression of how one might conceive of federated influence and belief updating among multiple agents, where each agent is associated with a particular subgroup, such that certain agents inherit beliefs from other agents, even though they’re actually looking at very different kinds of data of different part of the world. It’s interesting to think about what would happen if you had lots of graphs in this instance, a factor graph, that were equipped with shared markup blankets so that they could talk to each other and exchange. And we heard some suggestions about this in terms of implications for generalized artificial intelligence and belief sharing.
16:55
From a purely physics perspective, an academic perspective, what should happen is that this kind of system will find its joint free energy. And that just means, from the point of view of the physics, it’s going to invince a generalized synchrony. So everything is mutually predictable in the sense that free energy just is surprise. The average surprise is minimized, and everything is mutually predictable. So we’re looking at a kind of harmony that is a free energy minimizing solution that can have many reasons. Here’s a reason by my friend Takua, in terms of bathing filtering, that ensues from this kind of organization as a model of social intelligence and communication. So I’m going to now just run through three different levels that we’ve been focusing on in terms of active influence, learning and selection.
17:55
First of all, we’re focusing one particular aspect of having paths at hand, or having a particular focus on paths, and the ability to now think about the way that we prescribe endpoints of these paths. So, from point of view of a physicist, you would normally describe a path in terms of the initial conditions in which you prepare a system and the endpoints, and then you normally worry about the distribution over paths between the two. And indeed, that’s where the variation of theology from which Feyman came, from the context of quantum electrodynamics. But from our point of view, it was interesting opportunity now to think about specifying the endpoints of paths as intentions or as intended goals.
18:50
So I sort of euphemistically described it in terms of if I know that I’m here, then therefore I think I should go there, but only if I know where I am going. And we can derive a very simple, a very effective kind of backward deduction from this sentiment, cartooned here in terms of if I know all my allowable transitions in this context, and I know where I’m going to end up, then I know all the states as I back up in time that afford me access to the final state. And if I know that I know which states to avoid. If I know which states to avoid, I can work out how close am I to my goal. And if I’m here at this distance from my goal in time, then I know where not to go next.
19:48
And what we can do is we can just simply put that where not to go next into the, or add it to the expected free energy with a large negative number and preclude directions of travel that I know preclude finally pertaining my desired endpoint or my intended endpoint. And this can be written down in terms of logical or adaptive inference very efficiently, affording very deep planning, which is basically not choosing the best route to a design endpoint, but really avoiding any states that preclude the HGD up at that endpoint and illustrate this kind of putting inductive constraints on active influence based upon the exceptional information gain, expected utility, I. E. The expected free energy. And that hasn’t changed now with constrainting these inductive constraint beds. So the obvious one is getting to locations in some complicated maze that we’ve already seen examples of this.
21:06
So we start off with exploration, using the expected free energy to maximize our information again about the parameters structural of the unknown world, usually leading to a deterministic and base optimal in the sense of optimal basing, design, interaction and navigation of my environment, covering all options, learning where I can and cannot go and once I’ve done my effectively planning to learn in the sense I am choosing those plans that have the greatest success. Again, why have I done that? Well, I can now learn to plan and find the best routes to my intended goals, and I’ve illustrated that here in terms of a couple of examples, just to make two points. The first point is that there are two kinds of ways of specifying good paths.
22:03
So the prior preferences, or the constraints or the cost that we are used to that underwrites the expected free energy, or certainly the risk component of the expected free energy can be regarded as basically constraints in terms of which parts of space I do not occupy. So they put constraints in the path that are in play throughout the execution of that path. And this should be contrasted with the notion of an intended goal state indexed by h in the previous slide, which is just about the end point where do I halt? And I’m illustrating that here. So under the same intended states here, I’ve got some strong constraints, prior preferences here for not thumbspressing black parts of this maze, and weak constraints.
23:03
So just to illustrate the different kinds of route planning that one can evince from this combination, or putting inductive constraints on this is sophisticated inference, showing there is an interplay between preferences described in terms of constraints and preferences described in terms of intentions. The other point I wanted to make is it’s interesting to note that constraints we define inactive inference for good reasons in outcome space. Those good reasons are simply if you haven’t yet learned your generative model, there is no state space to specify your goals. But if now we are sufficiently learned or developmentally mature, and we now have a generative model that frames and scaffolds a state space, we can now instantiate some instructional set or some intentions, or specify them from a high level of a generative model in state space.
24:06
So when I talk about inductive constraints on sophisticated inference, what I am implicitly saying is within this learned generative model I can now pick out certain latent states, hidden states that I want to end up in, and then leverage, in this instance the expected information gain and pursue sample inference under constraints to attain that particular goal. And this is an interesting distinction which speaks to different kinds of sentient behavior. So you can imagine certain organisms, indeed you can imagine thermostats complying with principles of control. They can be very expensive thermostats, and you can simulate handwriting and walking and the like.
25:03
But now there’s a bright line between intentional behavior based upon the endpoint of a particular plan versus those based upon prior preferences, say, a pride belief that I am walking or I am talking based upon my tinnitus attractor orbit in my joker bomb. We wanted to go back and revisit. An illustration of free engineerization in real neurons in vitro on glass was published in Neuron last year that it was claimed showed sexual behaviour because they learnt to play pong. So Bret Kagan and colleagues in Australia basically put electrodes into a dish of neurons, supplied it or stimulated some electrodes, endowing this little in vitro neural network with information about configuration of a gain from a pond in a computer, but also have other electrodes picking up signals from the neurons that were capable of driving a paddle.
26:22
And the fond hope was that this in vitro neural network, biological neural network, would learn to play pond. Why? Well, because whenever it missed the ball, either any feedback was withheld or random feedback was supplied. So under the premise that the neural network was indeed self organizing, under the energy principle, it wouldn’t like random, unpredictable or surprising input. And therefore the only way to avoid that was to hit the ball back. And indeed that’s what it did. But the question is, to what degree could we find any evidence for intentional hit? Did it intend to hit the ball? Was it just avoiding constraints implied by the preferences, to avoid surprise, namely avoiding? Not anymore. So we simulated this in vivo neural network in silico.
27:19
In vitro neural network in silico to see how quickly we’ve learned a pom and compare it with the actual learning and what we did. So initially, this is the first 500 exposures, and what you can see whenever the ball hits the bottom row, there’s a sort of white noise, visual white noise, that the agent cannot predict, and therefore will always be irreducibly surprising. But as time goes on, it does actually engage in a few rallies where each rally entails a return of the ball for between two and six returns very much like the real neural network. But then we continued the simulation and saw this kind of behavior under this inductive inference. So after about 500 exposures, this is a single epoch with just 500 data points, it starts to learn to gracefully play pop.
28:33
And in so doing, both minimize surprise in terms of avoiding this random input. But also in this instance, we actually told it. At this point, hit the ball, leaves our particular state, basically got be in contact with the ball, because it had learned a suitable generative model, illustrated by the likelihood mapping and transition dynamics of the previous slide. So the neural networks never showed this behavior. They only got up to here, they never went there. So in Argento, they were sentinel, but they were certainly not intentional. So you could say that they showed control of the authors, but not planning as inference of this inductive sort or intentional sort. Good. Exactly the same kind of learning and active inference deployed using the same code and the same inversion routines.
29:39
But in this instance, in terms of the tower of annoying program, a little game where you have to move these balls around on pillars to match a target configuration, and you can make it easy or difficult, depending on the number of moons, to get to this arrangement. For this arrangement, in this instance, there are four moons. It can learn the model in terms of the likelihood mapping and the allowable transitions of all the different arrangements of these balls, and then we can switch on this kind of inductive inference and in so doing, move. So this was sophisticated inference with just one step ahead. And it’s not too bad, providing you enough time to work towards the desired or the required arrangement of balls. And it can even solve problems up to four moves ahead on occasions.
30:33
But as soon as you switch on the seductive inference, it gets 100% performance up to nine moves ahead, it’s actually looking. In this instance, 64 moves ahead. But you go much further in the future, should you want to. Active learning in a particular context, in this context was established by thinking about how one could deploy this mechanic, this kind of basic mechanics at scale, in terms of distributed cognition, ecosystems of intelligence, and implicitly federated influence. So my focus in this example is going to be on communication. How do you share beliefs among this federated and deep graphical structure where agents are now subgraphs within this generative model of itself and the external market blanket. So then, sort of, I’m going to use a game, hide and seek.
31:41
So imagine there are three daughters or sisters playing hide and seek with their mother and their mother’s moving around trying to hide behind this and that. And the key thing is, each of the sisters can only see a limited field of view. They can move their eyes around, but they can only see a limited horizon. And that horizon is distinct for each of the three systems. And the idea is, can we see or can we demonstrate the improvement in active inference, or the resolution of uncertainty and implicitly free energy if this sister can hear her sisters. And we equipped each of these agents with visual and auditory modalities. Visual modality had some high resolution fovinal representations and low resolution peripheral vision, in addition to proprioception where they were looking, and auditory, basically the sounds emitted by the other two.
32:46
Out of any given sustest herself, a very simple generative model. I’ve just written down the first few lines of the Matlab code describing the gerative model for each of the three sisters. And then basically this is what happens at the top. This is what one particular agent would actually see through her various visual modalities. This is what was being presented, what the agent could see, if she could see everywhere. And this is what the agent thinks she is seeing, noting that she can only actually see a very limited field of view at any one time. So our question was, what would happen if each sister brought to cast their beliefs by uttering the location of the mother? So just saying a word that indicated their posterior belief about the location of the mother.
33:48
And we simulated that simply by using precise and imprecise likelihood mappings to the auditory modality, and illustrating the results of being able to federate belief updating through communication, through articulation, through language, if you like. And I’ve done that here. We did that here in terms of beliefs about location. So these are the beliefs of the three agents, with and without language or communication. The belief of the first agent is very precise, because she could actually see her mother, but the other two agents couldn’t. But what they can do is listen to what the first sister is saying and resolve their uncertainty for very precise beliefs, even though they’ve never seen mum at this stage.
34:37
And we know that because when we remove this belief sharing, there is a lot of uncertainty about the location of the mother until the mother is actually visualized, because that’s the only sensory evidence it has to resolve uncertainty about mum’s location. And one can score it in terms of the free energy differences. So that’s the setup that was motivated by an illustration of the utility of sharing beliefs amongst multiple agents. We had another agenda in mind, though, in setting up this particular game. The agenda was to demonstrate the importance of active learning. And I repeat, active learning has a very particular meaning in this instance. It’s treating the notion of updating parameters, or generative model, as a natural. And it is natural.
35:33
The probability of doing it should be in proportion to a submarinex function, the expected free energy of doing it, relative to the expected free energy of not doing it. So now we introduce the notion of the expected free energy of a parameter that is acted upon through an update. And here’s the update we’ve heard about the importance of heavy associative learning, inactive learning in discrete spaces. This is really trivial. It’s just the pre post celtic activity of posterior probabilities multiplied together and then that provides the update to the weight or the parameter of, in this instance, the likelihood mapping. And what we are saying is we can either update or not, and score the probability of updating in terms of the differences in the expected free energy of that parameterization. So what is the expected free energy of the parameterization? And it’s really simple.
36:38
It’s just the mutual information that is constrained by our constraints or by our preferences. I think this is a really important observation, because what it says is that all learning at the end of the day is just in the service of maximizing mutual information, not just on the light of the mapping, but also on the transition to mappings and anything else that constitutes the Gerrito model. And of course, the estimate of a mutual information, certainly in dispute. State models is where each column of a mapping or morphism has one element and a zero everywhere. So it entails sparsity. It just means that the expected free energy of learning is just the drive towards the sparse, non redundant, high neutral information, high efficiency mapping, this instance a likelihood mapping, or more conservative, it’s not quite that simple. So there has to be a constraint.
37:38
We actually heard about explicitly the constraints on the sparsification or the maximization lot of mutual information. Those constraints in active influence are just supplied by the constraints that shape the kind of states that you would expect to find this particular system in. So when one does that and works through the maps, you have a very simple room for updating.
38:05
And what basically that does is it means that I’m only going to update if this is the right thing to do in terms of maximizing the marginal likelihood of my model or the free energy of my model, and if the mutual information, constrained mutual information is increased, if we now to go back to those three agents, those three sisters, and start off with a random likelihood mapping between their concepts, their posterior beliefs about hidden state of the world, and their articulation, their belief sharing, so that they know no language. And then we just let them listen to each other. Under this particular kind of active learning, then what emerges is a high mutual information that is shared. It’s shared because they’re all jointly producing the same belief.
39:10
So the only predictable exchanges that afford a high mutual information mapping are a shared acquisition of the same language. So the words, the articulations producing the auditory sensations mean the same thing. And this is an emergent property. This language is an emergent property simply out of maximizing the expected free energy of the model parameters or the mutual information. That’s cartoon here in terms of the convergence of parameters and reduction of free energy due to influence of learning, and the slow increase over four to 500 exposures in terms of nature information. And so, finally, moving on to a similar idea, but this is not in terms of updating parameters, but actually updating the structure of the model itself.
40:10
And what that leads to is the notion of active model selection, where we use something that’s underwritten statistics for model comparison for decades and decades, basically on the savage Dickey ratio. You may recognize this as externalization in terms of basic model reduction. And all we’re doing here is saying that there’s an acting thing, and the acting thing, do we equip my generative model with another state, another factor, another level to a given hidden state? And answering that question might say, well, what happens to the expected free API to the marginal likelihood of the model evidence? And under the assumption that the two likelihoods, sorry, the two likelihoods, are the same, not under that assumption, but under the assumption that we have some particular prior about whether this new observation warrants is likely or not.
41:21
I can then evaluate the relative probability of two models and decide whether to update or not by comparing the model evidence for this new observation. By comparing two models with and without an extra latent state or level to a latent state, a new path, or a new factor of latency states, each equipped with the nephrosis. It’s just one path identity matrix or stationary mapping, and two levels to it. So I’m illustrating that probably simply’s application here, which is just in a static context, just trying to classify or carve nature of its joints in terms of identifying how many species are there. If I was an ecologist or an evolutionary theorist, or in this instance, how many species of handwritten digits are there in terms of the style in which I can write any one particular digit.
42:31
So all we’re doing here is only updating our model by adding a new style for any given particular digit, if this condensation is met, and that’s what’s meant by active model selection, and we can control that with a species discovery, prior plays the same kind of role as all the stick breaking processes and, say, chinese restaurant processes that you would find in non parametric based. A little bit simpler in this instance. And I can accumulate thousands and thousands of observations of handwritten distributes here the youngest data set, and work out how many different styles there are. And once I’ve done that, I got two factors. One is the class, the number class, and the other one is the style. The style class. And here I think there are 256 different weights of variety, certain numbers, other numbers, only about 50 weights of items.
43:32
That’s actually number one here. And doing that, you can, having grown your model, kind of benzal model expansion based upon this active selection, one can then look at the learned or the acquired or accumulated information geometry, by looking at the information that’s approximated by KL divergences between different classes and different styles, and proceed in the normal way in terms of clustering, in the sense these different map numbers finally taking the same notion, but now into dynamics, where now the way that you grow the model, the way in which you deploy this active model selection, you’re accommodating or making a decision in the face of any new bit of data. Do I augment the model or not? If I do augment model, how do I augment it? Do I have another state? Do I have another path to any given factor?
44:46
Or do I actually add a new factor in transparency, simplify that and develop a kind of structure learning that depends upon the sequence in which you present data for a simulation, for the agent to make a decision about whether to grow or expand the model or not, with a relatively simple curriculum. So, to summarize this in narrative form here. So if this is the very first thing I see, I’m going to create a lightning mapping with a single state and populate it with initial derivation, a count, namely concentration parameter of a symmetric derivation, a distribution. Then for every other observation, I’m going to consider an additional state, the last factor, provided there’s only one path, and that path is by design a stationary path, a path that we just remain in the same state. Otherwise, consider an additional path or factor.
45:43
And if I’m putting a new factor in, then it’s going to again clearly have just two states with one stationary path, because the third state is a state of the previous factors that is required to link different factors together. And when we use that kind of structured teaching, really because you have to be very careful about the sequence in which you present the successive data points that are being assimilated, not too careful, because it’s exactly in the order that the dynamics of the real world represented. But still, you have to actually be careful when you’re simulating this. We can now do structure learning of a kind that could be, that is a very elemental disentanglement of sprites here, and yes, the sprites themselves, summarized here.
46:45
So in this particular example, the world could contain one of three different kinds of objects, and these objects could move around just to make things interesting, we actually equipped the agents with prior preferences for locating each of the three different kinds of sprites to different quadrants. Following the work of colleagues zapped and Kent, and crucially, the locations. The preferred locations of each of these objects were different. Each object can move up or down or sideways or both. But the agent didn’t go. The agent was completely naive about the MDP structure. The market decision, or partial market decision process was never actually specified by hand. It was automatically rode as fact. Immediate data that had been generated from a process that had already been learned.
47:51
And what it can do, or what it should do, is basically learn receptive fields for vertical and horizontal positions and the map of preferred locations that will ultimately cause it to draw these sprouts to the locations. And indeed it does using this. So this is the learned auto assembly itself created structure learning. In terms of the likelihood mapping, it’s all been concatenated for visualization and the different transition dynamics for each of three factors. It’s known there are three factors. Basically these three factors are things can move right and left, or they may not be moving, or they can be moving up and down or not moving up and down. And there can be three kinds of things that are moving left and right or up and down.
48:51
And it’s also learnt the preference mappings here, this is called the number of times each of these areas, each of these locations were revisited. I should say an important part of this kind of structure learning is after a time, the agent auto didactically makes moves to fill in the gaps like motor babbling, based upon those actions that maximize exceptional information about the parameters. So once it’s got the structure in terms of the number of factors and paths within each factor. So number of factors here, number of levels for each factor, number of paths for each factor over the columns here. Once it’s got the structure, then it can sample the world in a way to fill in the tensors on the basis of informed epistemic moves that are chosen on the basis of the extracted energy, or I repeat, the extracted information parameters.
49:54
And it learns exactly the right receptive fields and like mappings. And this is the final slide. It just illustrates the kind of learning that you get and events is something that you see at least twice, if not more times, throughout the presentations during the workshop. This systematic progression from exploratory behavior to exploitative behavior. So this is the number of trials during this epistemic foraging, during this, if you like, motor babbling, that show that initially it requires, as one might guess, given this is a sort of nine by nine visual scene, it requires about 81 exposures to start to fill in all the important gaps here, during which time accepted information here is very high for each of the three sprites here, with the evidence low bound here going up and down during this exploratory phase.
51:06
But after it’s learned and notice it has very precise beliefs, it’s very confident just to pick up one presentation. It’s very confident. It has a low entropy distribution over policies and over where to move the sprite on each time step during the exploration phase, because it knows what it epistemically has high epistemic affordance and therefore makes very confident epistemic moves initially. And then as it becomes familiar, the acceptance information gain falls, it starts to lose some confidence in terms of the precisions and throws down to a reward based exploitative pattern of behavior and just maintains the sprite of its preferred direction and becomes bored, presumably. And that’s it. That’s a brief whirlwind tour of what me and my friends have been doing in the past year. I’ll close with Einstein lock. Evidence is basically simplicity plus accuracy.
52:17
In other words, everything should remain as simple as possible, but not simpler. Just to recapitulate that in terms of planning’s influence and the expected free energy, the important thing is not to stop questioning curiosity as its own reason for existing. Indeed, I would argue that existence just is the expression of curiosity. So that just remains, again, for me to thank all my collaborators. Their affiliations are shown along the top here, and I repeat, most of you in the audience anyway, so you know exactly who you are. I’d like to thank you for your attention, but just take this opportunity just to thank Tim and the organizers for this opportunity. Thanking me for attending, I thought was quite incompetent yesterday because I was really enjoying myself, sitting here admiring everything, having a cup of coffee, and smoking the k abandon. So.
53:37
Thank you very much for that talk. I’m interested in this adaptive structure learning that you’re doing, and I wonder if you could speak a bit on the idea of I’ve come across the fact that disentanglement doesn’t always necessarily give you strong generalization abilities, and I wonder if you could perhaps talk a little bit about how you might necessarily be able to do adaptive structure learning, but making sure that you’re getting a real causal structure as opposed to a furious structure.
54:20
Excellent question. I think just a broad answer, and I’m sure that people will come in with their perspectives on this, the broad answer is that this kind of structure learning based upon building a model from scratch is quite fragile. And it’s certainly not the way that biotic or biometric, at least structure learning would work. You’d normally use basic model reduction and start off with something that has the right kind of structure that’s usually overexpressive, over connected. It’s like a baby’s brain, and then reduce it in the right kind of way, fit for purpose for this particular lived world. But you could still ask your question, what happens if I go through my period of base model reduction or neurodevelopment in one world, and then the world changes dramatically? How do I then adapt to this new world?
55:23
And then one might argue that we need some kind of mixture of recurrent basin model reduction and basin model expansion. And indeed, if you’re a biologist, you might argue that’s exactly what we do in terms of various new developmental cycles, but also what we all do every day in terms of our sweet wake cycle. We do our basin model augmentation during the day, require excessive and exuberant associative connections through hepasticity during the day, and then during sleep we do the basic model reduction. There is no accuracy term to worry about. So maximizing marginal likelihood of the elbow or minimizing variation free energy just is minimizing complexity by removing redundant model parameters. And just I forgot to mention that there was a really interesting presentation.
56:20
It may be penaltime presentation where somebody was asking the presenter, well, if you’ve removed the environment, how could it ask questions about itself? And it did strike me that was like putting it to sleep, that you can still optimize a model in the absence of any new vertical data, as we’ve known since the inception of the objective wake sleep algorithm, your view through the lens of structuring and beta moral selection, this is a necessary part. You need some offline period in order to minimize the complexity. So you’re not worried about maximizing the accuracy. You would imagine that just having baby model expansion in and of itself, by itself, from scratch, from, if you like, an embryo, a single cell just will not work. I think you have to put it together with basic model reduction.
57:21
However, if you wanted to do it, then you’re absolutely right. It would just learn that world structure that you exposed it to. And if you change this, it would have some optimal and sets of model, evidence generator model. And this I think places strong emphasis, I repeat, on the curriculum of the data that you present. Because the order sequentiality, the ordinality of the data is absolutely crucial for learning the dynamics, and much of the disentanglement rests upon the dynamics. So if you don’t present things in the right order, it will never learn the right kind of model.
58:08
And in so doing, this order really matters, very much like curriculum, learning in machine learning, or in education, at least you sort of freeze what you’ve learned up until now, prior, if you want to introduce a new level, a new factor, or a new path, so you can’t go back and undo what you’ve already learned. So again, very much like education, it’s very important you educate and teach in the right order and build upon what has been learned so far. So that would be my intuitive answer. But I repeat, I’m not sure this kind of basic model expansion is going to be sufficiently robust to be a generic tool. Might be, perhaps, Chris, but we’re going to try.
59:08
So, thank you for the presentation. In Jackson presented the universal genitive model. From what you showed in your presentation, there was essentially two ways to go about it. Either you go from practically a bolt of a generative model that grows through structure to what it needs to become, or you have a massive gen model where you some kind of attention, you just threw it away at the end of time. Then you showed the active learning language where it seemed like you were starting something. Collaboration, federated learning and activity bring your agents to coalesce on a shared model. And therefore we could assume that you could use this to determine the kinds of separation of a part of universal generative model, to make kinds of agents for kinds of purposes.
01:00:07
So what approach do you think would best to get to the universal generative model relating to these different approaches? And if you worry that you’re learning, how would you structure a system that could get us, after going to the right kind of agents, the right kind of witnesses from the universal genesis model.
01:00:30
In the context of, if you like assembling federated models, large structures, what you are basically asking is, how do I plug in a new model into an existing ecosystem? So again, this very much speaks to what I was trying to intimate before, that learning the new model de novo is probably not the way that sort of biometric systems self organize you. I can’t see you, I recognize your voice. But if it is what we’re talking about here, as you will know, of course, the circular causality implicit in niche construction, cultural niche construction in the context of language that enables that kind of belief sharing and language learning that I illustrated. Now, notice that in the illustration of that belief sharing. The agents had pre formed or learned generative models of their lived world.
01:01:40
All they had to learn was the mapping between their beliefs and what they said, how they share those beliefs. So this speaks to a general theme that you’re sort of scaffolding a particular kind of learning in this instance. And I think this is reference to your question, learning about the meaning of other people’s utterances or messages or cues or language in relation to my own beliefs. So the belief sharing rests upon or that particular kind of active learning is a way of literally plugging in or integrating one generative model into a network or a federation or an ensemble of generative models.
01:02:30
And the pace at which you pluck it is going to be very interesting, because if you come back to that sort of circular structure, that centripetal deep juncture model with the clock rotating around, then certain agents will be quite slow agents, and other agents will be very fast agents. So it’s got to find its place not only in terms of being able to share beliefs with its siblings and either assimilate data that it can see in terms of likelihood matters and discern and choose the right. I think, Bert, use the word subscribe to the top down empirical prize from the level above. So the belief show we’re talking about at the moment is horizontal within an eleven, but it’s also got to find the right level.
01:03:26
So if it’s a very slow agent, if it’s the kind of agent that does, say, climate forecasting, then it’s not going to be able to talk to the kinds of agents involved in traffic flow maintenance in smart cities. So part of this active learning will not only be, but the active learning part, I think will be very important. Automatically learning the way that you exchange with your conspecifics at any particular level. But possibly the Beijing model comparison will be very important to finding what level you as a part of this subgraph, or you as a subgraph, and you could be artificial, you could be real, you actually fit into this distributed kind of message asset thing. Is that the kind of answer you were looking for?
01:04:23
Yeah, I can imagine that based on what you’re suggesting, we could just split the model into see how other performance learn the proper breaking breakpoint for the model to book the.
01:04:41
That’s certainly true. We have seen an example of this before in terms of convergence depends upon the precision. So another phenomena here that would, I think, askew having to dissect out or reposition an agent would be the observation that under basic monospection and parametric learning, then because of this observation, that the joint free s energy of if you like the ensemble and the new individual is when they are mutually predictable, then there will be a generalized synchrony, which necessarily entails that there is an isomorphism between the judgment models that the new agent can see of the ensemble and the new agent. So there’s a convergence. Your new agent will, the child, if you like, or novice, will come to install the structure, the parameters of the environment in which it finds itself.
01:06:00
But of course, that environment is actually another agent, in fact, partly sub agent, of a larger ensemble of agents, provided that the agent has more precise beliefs. So the degree of convergence, I think the degree of convergence of agent to environment as agent depends upon the relative precision of the beliefs. In discrete formulations, those precisions are encoded literally by the number of deri counts. So a baby agent will have very small derision counts. So as soon as you start adding counts, their relative probability has changed very quickly. So they’re very impressionable. An old wide agent that’s been around for a long time will accumulate lots of traditional accounts and add in one or two here or there will make very little difference to their belief structures and their morphisms or likelihood mappings.
01:06:57
So depending on the rounding amount precision of the two agents, the questionable agent will come to adopt that of the teaching of the wise agent, or the owned or the mature agent. So it may be that an agent can actually adapt to any particular scale or any particular exchange, provided it is sufficiently impressionable. But we are talking here. Sorry, just a nod to Connor’s work when he was talking about symmetries in terms of learning rates. You can always interpret a learning rate in terms of precision. So what he was talking about in that instance was a precision there articulated in terms of how quickly do you accumulate your precise, conventional accounts. So that symmetry versus asymmetry is really important in terms of whether you get this cooperation or defection. But more generally speaking, it’s almost cooperation moving towards the synchronization marathon.
01:08:00
But the degree to which any one person moves depends upon, or agent moves depends upon the relative precision of their beliefs relative to the notice. All we talk about here is just parametric learning, though it’s not structural. That’s probably a more vexed problem.
01:08:27
I have a general question. Hi, Carl. So this is just more of a general question, but last month, as I’m sure you’re aware, a research team in Japan at Riken published a paper that offered that they had achieved experimental validation that the free energy principle is indeed how neurons learn. So I’m just wondering if you have any thoughts to share on that in regard to that.
01:09:03
Yeah, I think that must be the work of Takua, who I mentioned during the presentation. So the work on the in viva neurons playing pong, that was with Bret Kagan and colleagues in Australia. The japanese group have done a lot of these foundational conceptual work using in vivo neurons, taking a number of steps, providing empirical evidence that indeed, agents were behaved as if in vivo neural networks behaved as if they were minimizing their free energy.
01:09:51
But the most definitive account of that, I think, is available in the paper you just mentioned, which was a beautiful piece of work, a little bit involved, if I remember correctly, in the sense that what they had to do in order to assert that these in vivo DNA particle neural networks were indeed minimizing their variation free energy was to first identify the generative model that these neural networks have of their sensed world, because the variation free energy is only defined in relation to a generative model. More specifically, free energy gradients require a generative model. So that’s something which is often overlooked in terms of applying the free energy principle to biological systems. And indeed, your people thinking about the application of the FEP, or active influence, to computational psychiatry.
01:10:51
It’s not easy to work out what genetic model anyone’s actually using, but they’re able to do that for these neural networks just because of the simplicity of the way that neurons talk to each other, and by appealing to artificial neural networks. So they were able to take canonical forms from biologically plausible neural networks, deep neural networks, or recurrent neural networks, and then work out what the implicit generative model was actually state space model, if I remember correctly. So that they were able to, if you like, identify or reverse engineer the gerative model. A very simple world was basically, yes, no, or your kind of world is the world in this state, or is it in that state?
01:11:44
But in so doing, they were now able to look at the empirical inputs and outputs to this in vivo neural network, infer the geriatric model and its parameters quantitatively, and then the validation enabled them to take those quantitative parameters and precision parameters, and then work out how quickly it would learn and how it would respond to perturbations to various parameters in the model, or changes in the parameters of the model that were associated with particular phrase interventions. So the remarkable thing, which is not obvious, and Eden’s remarkable, they were able to get it into the literature so easily, I think. But the key thing they’re able to do is basically say, I now know how this in vitro neural network will respond in the future.
01:12:50
I now know how it will learn, because I’ve completely parameterized its generative model, and that is under the assumption it’s going to minimize its expected energy in the future. I can now determine its learning rate, what it will learn, how quickly it will learn, and how that learning will be perturbed by various interventions. And that’s what they use as a validation of the free energy principle. So, lovely piece of work based upon at least a decade’s worth of work. It may not be a parrot of paper, but I do know, because I know the first author, and how much is invested in that.
01:13:43
So the beauty of bridging festival is this kind of commitment to Baylock’s landlord to lead to all this lovely machinery. But lots of us are trying to fail close to it, from the algorithm to the computational barrier. So how do we implement this at scale, particularly in artificial intelligence and so on?
01:14:03
I just wonder what you feel about that.
01:14:04
So you can think about that in terms of how the brain works, and you can also think about it about how we do it in silicon. And those might have different suggestions about the implementation. My general question is that kind of staying close to the hardware and.
01:14:19
The constraints something that will give back.
01:14:22
To the algorithm level, you feel separate, so the base optionality is somehow free from the implementation? What is that discourse between two? So just to give motivation for this.
01:14:32
Question, Jeff Vincent recently said that he.
01:14:35
No longer considers the brain source of inspiration for AI and fabrication. If the brain do fabrication, we should no longer consider neural systems as somehow inspirational systems.
01:14:56
It sounds though you want me to answer Jeff’s challenge there also, Jeff is responsible for the notion of morph computation recently, which was speaking exactly the opposite direction, I think. I’m sure you’ve got your own views on this, and I’m going to ask, I’m going to pass it back to you to articulate my view on this, is that the brain as an inspiration, the commitment to biometric implementations is a principal approach, because we are the existence proof of the emergence of this kind of self organization, and it is scale free. I wouldn’t be here without my parents. My parents wouldn’t be here without the welfare state or a culture. Culture wouldn’t be there without the biosphere gear, and also myself wouldn’t be here without me.
01:15:56
So it’s important to remember that you cannot just build Agi in silicon it has to evolve in a context that itself complies with the right principles and so on and so forth. So I’m pretty sure that the principles are generic, and the principles do emphasize being attendant to the neuromorphic constraints, in the sense that the whole point of building a jerative model is that it has some kind of structuralized morphism to the world that you’re exchanging with. So if you pursue Jeff’s argument to the limit, then what he is saying is, well, if I want to build some kind of intelligence that is not able to exchange or assimilate or accommodate biomimetic intelligence, then yes, I can free myself from the constraints of neuromorphic imperatives or biomimetic imperatives. But I don’t think that’s a workable agenda.
01:17:15
Putting it the other way around, is it likely that in the future, that the ecosystem as we know it, and of which we are part, will no longer exist, and it will have been replaced by encircular intelligence? If the answer is no, then that means that the kind of intelligence that you’re having to build has a degree of generalized thinking, the eyes, the morphism in time and in space, with us, with you and me. And if that’s the case, then it has to be biometric. And therefore, and the structure matters. The structure of the graph, the message pattern, the timing, the temporal scheduling, all of that matters to the spiritual regulator theorem, provided you remember that it has to actually interact. It is open to the ecosystem in which it is embedded. So that would be my pushback against Jeff’s challenge.
01:18:20
Do you have pushback, or do you agree with it?
01:18:25
I don’t know. I’m sympathetic. I guess the end product of the AI system and how you get there are kind of different things, actually. You can take propagation route to a system, and then how does that affect the end structure that you achieve? I don’t think, in principle, that we couldn’t get something that was biometric by more. In lots of the processes that we get in biological systems, for example.
01:19:00
Do.
01:19:01
We have to have a full evolutionary process before we get truly has generalized synchrony with a biological agent? I’m slightly doubtful about that, but also, I think he’s maybe simplified it too much. I do think sitting at the boundary of the competition, we’re going to learn a lot. So, one thing I worry about is that bay in autism has to be under constraint. So optimality of bay is constrained by the medium which is implemented. And that puts out important implications and even our own behavior diversionality.
01:19:44
Just to pick up on that point, just again, try to link it with discussions of controllers inference, the distinction between having sort of a biased model of the world versus a little model of the world. I think your last point really highlights and foregrounds that issue. I would argue with my hat, as a data scientist, as a modeler of brain imaging data, I would argue that Bayes just is the right way to implement constraints. So normally, you only recourse to Bayes in the context of imposed problems that are only solved through the imposition of constraints. So Bayes is a mechanics of constraints, and indeed, the generative model in the free energy principle just is an expression of the constraints on the kinds of states that this particular particle or person can occupy. They are characteristic of that person. It is all about constraints.
01:20:51
And just, again, just trying to pick up on some really interesting themes that also, I think, hits you in the face when you take Dontleman’s perspective on the free energy principle as dual to the constraint mathematical principle of James. But notice the emphasis on the constraints. So, from my point of view, the constraints on the max end principle just is the journey model that says it has to be like this and it has to interact like this. So what that means is the free entry principle just is a constraint principle where all the interesting stuff is in the constraints, because the entropy is just what it is. You can easily measure that. We’ve seen that lake under gaussian subject numerous times in the presentations. It’s all about the structure on the Janji model that affords those constraints.
01:21:50
The link I want to make is to the rate distortion theorem, which again I see is just another instance of James’backswing principle, or the free energy principle, beautifully articulated by the presenter. The rate of distortion just mapped very gracefully to a bipartition of the marginal evidence, or likelihood model evidence into accuracy and complexity. It’s all the same thing. And yes, you can call it basic. You could actually articulate the engineer principle just by using the language of James or the language of Shannon. In terms of red distortion theory, you can articulate and use engage theory. So if there was any sense in which can you wander away from Bayes, I would say absolutely not. Is there any sense that you can wander away from neuromorphic implementation, then that’s, I think, a more subtle question.
01:22:53
If it’s an implementation of neuromatic computation, I would say, yeah, absolutely. Can you wander away from neuromatic implementation? Sorry, processing. I would say no. I would say no for the reasons I just articulated. Can you give to the next people. Hello Carl, I was wondering, do you imagine a world which minimizes only the expected free energy and not vibrational? Do you imagine a word which minimizes only the expected energy and not the.
01:23:36
Vibrational free energy.
01:23:41
Because your entire presentation was in this direction? No. From a physicist point of view, the expected free energy is just part of the overall free energy. But the way it was unpacked in the presentation was just to make it clear that the expected free energy sort of plays a role of a prime in physics. It sort of comes out of the pathogenical formulation as a probability distribution over the active states over the actions of something. All particles are detective of many random fluctuations, but it cannot be realized, or indeed computing computed in the absence of hats and implicit distributions over everything else, including the external states and the internal states. So expect to be able to enjoy your thoughts. It’s not the only thing.
01:24:55
So that’s about five business so far.
01:25:01
And, well, I’m looking forward to who won the poster. Tim? Did anybody miss the boat?
01:25:12
Yeah, Mustang collected all the boats, so.
01:25:15
He knows I meant the boats. The boat. Yesterday he was so worried about people missing the boat. Everybody knows the boat. I was sitting very worried in the UK. Thank you very much again, it’s been amazing. I’ve sat here and I have been thanked for sitting here gloomed to the screen, listening to all these ideas. I’ll just respect me. Normally I can summarize 99% of what’s been said. What has happened in the past couple of years is I can’t anymore. This whole field is moving in so many different directions. I think it’s almost impossible for any one person to have an expert overview on what’s going on. So more and more I am finding. I am learning stuff.
01:26:10
So I just wanted to say that when you get to my age is a bit Friday, but I suppose that speaks to the importance of the next generation of people coming in and taking up the mantle of pushing the envelope forward. And that pushing, I think, has been beautifully evidenced in the presentations and in the youth and fresh ideas that have been showcased in the presentations. I will finish because this is probably my last opportunity to say anything, but I repeat, Tim and your colleagues and the person and the people who’ve allowed me to and other guests to actually participate online. I just wanted to thank you for all of your energy and organization and good humour in being the architect of this workshop. So thank you very much.
The convergence of two groundbreaking technologies is reshaping how we think about AI, automation, and intelligent systems, affecting every industry...
On 29 May 2025, the IEEE Standards Board cast the final vote that transformed P2874 into the official IEEE 2874–2025...
Explore the future of agent communication protocols like MCP, ACP, A2A, and ANP in the age of the Spatial Web...
Fusing neurons with silicon chips might sound like science fiction, but for Cortical Labs, it represents what's possible in AI...
Ten years ago, on March 31, 2015, I interviewed Katryna Dow, CEO and Founder of Meeco, to discuss an emerging...
We stand at a critical juncture in AI that few truly understand. LLMs can automate tasks but lack true agency....
VERSES AI 's New Genius™ Platform Delivers Far More Performance than Open AI's Most Advanced Model at a Fraction of...
Go behind the scenes of Genius —with probabilistic models, explainable and self-organizing AI, able to reason, plan and adapt under...
By understanding and adopting Active Inference AI, enterprises can overcome the limitations of deep learning models, unlocking smarter, more responsive,...