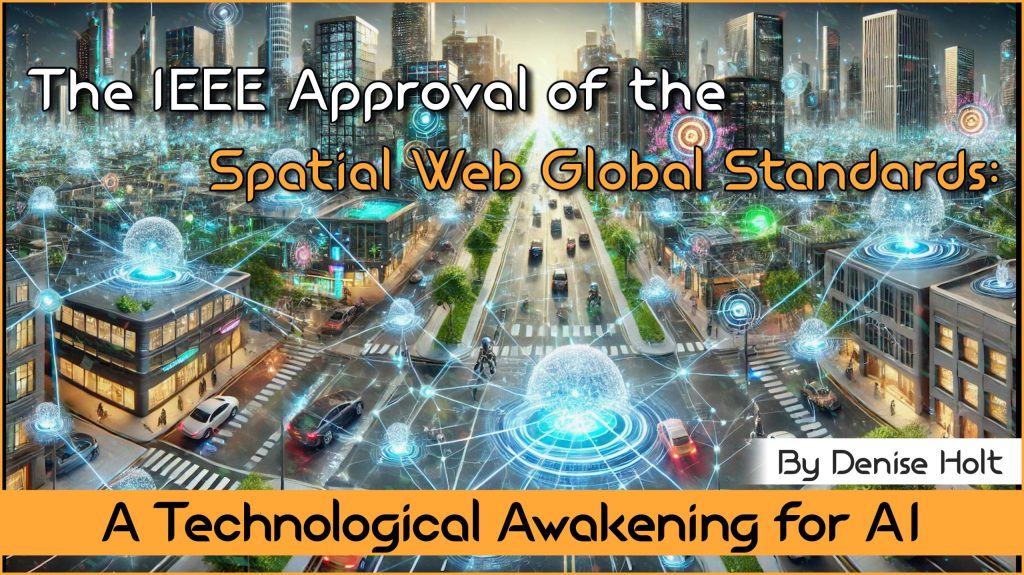
VERSES AI is transforming AI, shifting us towards First Principles AI, Embodied AI, and Decentralized AI, distributing AI compute to…
Dr. Karl J. Friston is a British neuroscientist and theoretician known for his contributions to the fields of neuroimaging and computational neuroscience, as well as being one of the most notable scientists in the field of Human Brain Mapping. He is also the Professor of Neuroscience at University College London and the Scientific Director of the Wellcome Trust Centre for Neuroimaging. Most recently, he joined VERSES AI as Chief Scientist, leading the development of their next-generation Artificial Intelligence – and what’s most exciting — a clear roadmap to Artificial General Intelligence — the holy grail of AI.
Dr. Friston is an authority on brain imaging and the most cited neuroscientist in the world due to his groundbreaking technical contributions and inventions which approach understanding the architecture of intelligent systems and how the brain processes information by relating them to biological design principles found throughout the human body and nature, itself.
His earlier work was motivated by schizophrenia research, where he made some truly groundbreaking technical contributions, developing statistical tools for analyzing brain data. Among these were Statistical Parametric Mapping (SPM) and Voxel-Based Morphometry (VBM), which led to his invention of Dynamic Causal Modeling (DCM), used to interpret the structuring of distributed systems like brain activity. From there, his work led him to become the pioneer of Active Inference and the Free Energy Principle based on the theory of free energy minimization, demonstrating how neural networks are optimized for efficiency.
Recognized as the #1 most influential neuroscientist in the world by Semantic Scholar in 2016, Karl Friston has received several prestigious accolades for his work in the field of neuroscience. Notable awards include:
He also has been honored with several prestigious lectureships, such as the 2017 BBC Reith Lectures, the 2018 Penrose Lecture, the 2019 David Marr Lecture, and the 2020 Goulstonian Lecture. Additionally, Professor Friston has also been elected as a Fellow of the Academy of Medical Sciences, and a member of the European Dana Alliance for the Brain.
Active Inference is a theoretical framework that describes how agents, like animals, robots, or artificial systems, can maintain their internal states and behavior in unison with their goals or objectives.
The Free Energy Principle (FEP) is the theoretical foundation of Active Inference. It proposes that organisms or agents maintain their internal states and behavior by acting in a way that minimizes the difference between their current beliefs about the world and what they expect to be true. In other words, agents try to minimize the surprise or uncertainty about their beliefs by mitigating the discrepancy between what they expect to happen and what actually ends up happening.
In the context of artificial intelligence, active inference and the free energy principle are used to explain how agents can learn and adapt to new situations, and how they can generate predictions and plan actions based on their goals or objectives. The idea is that an agent can use active inference to minimize the free energy and optimize its behavior in a way that is consistent with its objectives — which leads to more accurate predictions and results — the real goal of AI.
Dr. Karl Friston has been working on the concept of Active Inference and its application to artificial intelligence for more than a decade. In 2010, he published a scientific paper titled, “The free-energy principle: a unified brain theory?” in which he first introduced the concept of Active Inference, proposing that Active Inference can be viewed as a general principle of self-organization that is not restricted to the brain but can also be applied to artificial systems.
Since then, Friston and his colleagues have been exploring the use of Active Inference and the Free Energy Principle in artificial neural networking systems, developing methods and algorithms for Active Inference in machine learning and artificial intelligence.
Most recently, in a press release on December 1, 2022, VERSES Technologies, a cognitive computing company specializing in next-generation AI, and creator of the Spatial Web Protocol, announced the addition of Dr. Karl Friston, welcoming him as Chief Scientist, leading VERSES AI research and development through Model Based AI for Active Inference.
In the announcement, Gabriel René, Founder, and CEO of VERSES expressed his excitement, stating, “Dr. Friston’s breakthrough work in neuroscience and biologically-inspired AI, known as Active Inference, aligns beautifully with our vision and mission to enable a “smarter world” where AI powers the applications of the 21st century. As the originator of this principle, it is only fitting that Karl has a significant role in VERSES AI research and development all the way through their applied uses in product commercialization.”
Active Inference is a biologically inspired approach to AI systems. As a method for understanding sentient behavior incorporating the brain, cognition, and behavior, modeled after the design principles from nature for how the brain, nervous system and body act and react. Model Based AI for Active Inference enables us to overcome current limitations within Machine Learning/Deep Learning AI models, providing a realistic path toward Artificial General Intelligence.
Active Inference AI, the Free Energy Principle through Bayesian inference, allows a neural network to self-optimize through intake and continuous updating of new real-time sensory data, while simultaneously considering previously established outputs and determinations.
Past Decisions → New Input → Future Outcomes = Self Optimization and Self Evolution
A Self Evolving System evolves over time. Current systems are more like a machine. Neural Nets are “trained” to do a specific task. A self-evolving system is learning from moment to moment and upgrading its World Model — and thus mimics biology while also enable General Intelligence.
Within the Free Energy Principle (FEP), neural networks self-optimize through a set of mathematical rules, enabling next-generation artificial intelligence to efficiently learn, predict, plan, and make decisions.
FEP is perfectly suited to the programming language of the Spatial Web, HSML (Hyperspace Modeling Language), which enables computable context based on defining, recording, and tracking the changing details in physical and digital dimensions, social dimensions, meanings, culture, conditions, circumstances, and situations, whether geometrical, geo-political, or geo-social by nature.
As impressive as all the current AI models are, it’s important to understand that they are not truly artificial intelligence. Although very useful tools, they are nothing more than Deep Learning predictive machines with an effective ability to recognize patterns. There is no actual thinking taking place, nor will they ever be capable of it. They are unable to understand any of the information they are processing. And most importantly, they are not able to consider circumstances, nor do they possess the ability to compute context in order for the machine to gather any important details to actually affect an outcome or decision. These machines are all using sheer pattern matching probability based on learning patterns established from being trained on old data, with no real decision making occurring, no ability to form any kind of judgement based on inclusion of extraneous data, and no ability to account for or adapt to real time information changes.
In order to be able to move forward with the development of artificial intelligence, these artificial systems need to be able to understand things on an abstract level, taking into account all the complexities surrounding information intake that leads to decision making. When thinking about how this works on a ‘systems’ level, it’s necessary to be able to break those nuances and details down in a way that can be recorded and measured.
This is exactly what HSML(Hyperspace Modeling language) can do.
Through HSTP (Hyperspace Transaction Protocol) and HSML (Hyperspace Modeling Language), context is coded into every object — person, place, or thing, whether physical or digital, to define, describe, and categorize distinguishable and evolving traits of any user, object, activity, or protocol in any environment, whether real-world or virtual.
In the recently released white paper entitled, Designing Ecosystems of Intelligence from First Principles, established and written by Karl J. Friston and the entire team at the VERSES Research Lab, their vision for artificial intelligence research and development is laid out with the purpose of fostering a “cyber-physical ecosystem of natural and synthetic sense-making, in which humans are integral participants — what we call ‘shared intelligence’. This vision is premised on active inference, a formulation of adaptive behavior that can be read as a physics of intelligence, and which inherits from the physics of self-organization. In this context, we understand intelligence as the capacity to accumulate evidence for a generative model of one’s sensed world — also known as self-evidencing.”
The concept is centered around the Spatial Web ecosystem becoming a distributed network of intelligent systems, basically AI-s nested within AI-s, interacting and evolving with each other simultaneously in real-time, able to calculate decisions based off continuously changing context and data across interactions between all people, places, and things in any space, whether real or virtual.
Network structures are at the core of advanced intelligence. Whether considering any of the body’s biological systems — circulatory, nervous, or cellular structure, or any other species in nature, even ant colonies, there is a self-organization aspect to the network operation.
Quoting the white paper, “We argue that the design of intelligent systems must begin from the physicality of information and its processing at every scale or level of self-organization. The result is AI that “scales up” the way nature does: by aggregating individual intelligences and their locally contextualized knowledge bases, within and across ecosystems, into “nested intelligences” — rather than by merely adding more data, parameters, or layers to a machine learning architecture.”
Some of the top considerations outlined by the research team:
Next generation AI systems must be based off a world model, holding definitive beliefs regarding the state of that world, with the ability to adapt and evolve within shared narratives.
The Spatial Web (Web 3.0) becomes this AI world model foundational ecosystem comprised of digital twins of every space, both real world and digital, along with every object — person, place, or thing within it.
Model-Based AI for Active Inference within this new Network of Everything, transforms into an artificial intelligence organism, that within itself represents the ultimate generative model for scaling to super intelligence. Utilizing the HSML context programming, baked into each entity within this new internet, a new collective super intelligence emerges actively learning and processing real time information and situational cues to continually refine its understanding of all things in all spaces. This ability for self-evidencing and self-organization naturally cultivates a more and more sophisticated entity of shared intelligence between human and machine, truly augmenting the human experience, redefining what it means to be human.,
Current AI models — Machine Learning/Deep Learning AI, are akin to “black boxes.” They intake prompts and output data without any way to understand the pathway to the results. The process within the neural networking of these systems is random and obscured, so there is no control, no way to audit or tweak its underlying approach to its processing actions. There is no way to refine its tendency toward accuracy other than to feed it more diverse examples to include inside its comparison bank.
This is also very problematic when you consider the materials used to train the system are bound to be flawed with human bias and corrupt data.
Active Inference AI within the Spatial Web is a completely auditable system on every level, and the pathway to results is both transparent and controllable.
In regard to other ethical concerns, the AI Research Team at VERSES possesses a high awareness to the potential dangers in balancing between individual perspectives and the abuses of power, especially when considering the preexisting power structures within society and cultures which will inevitably co-exist within the Spatial Web.
In this white paper, the ideology is clear, “We believe that developing a cyber-physical network of emergent intelligence in the manner described above not only ought to, but for architectural reasons must, be pursued in a way that positively values and safeguards the individuality of people (as well as potentially non-human persons).”
They go on to further exemplify this point by illustrating how every person is considered unique with their own life experiences and their own interpretations of meaning accumulated along the way. Each person has a singular and special set of knowledge that is valuable to the diverse understanding of the collective. To enable complex, shared super intelligence, diversity of perspective is essential and must be safe-guarded and preserved.
The VERSES AI Research Team propose that, “AGI [Artificial General Intelligence] and ASI [Artificial Super Intelligence] will emerge from the interaction of intelligences networked into a hyper-spatial web or ecosystem of natural and artificial intelligence,” by way of Active Inference AI as being uniquely suited to the task of creating a nested network of shared intelligence systems within the hyper-space ecosystem of the Spatial Web.
Image by permission from VERSES Technologies
“AGI has been the dream of science fiction for decades, but it has seemed impossible for ML [machine learning] to get there… For the first time in history, starting now, we have the roadmap. AGI is now possible.” — Dan Mapes, President, and Co-Founder, VERSES AI, and Director of The Spatial Web Foundation
To learn more about the Spatial Web Protocol and the evolution of Web 3.0, visit SpatialWebFoundation.org
All content on this site is independently created by Denise Holt. If you enjoy this content, please consider supporting my efforts at patreon.com/SpatialWebAI
VERSES AI is transforming AI, shifting us towards First Principles AI, Embodied AI, and Decentralized AI, distributing AI compute to…
Updated - Over 150 New Entries for 2024 Q2 - Research papers, articles, videos, who to follow, and more! The…
VERSES AI has unveiled a groundbreaking framework that "sets the stage" for ASI, and could have profound implications for the…
In a panel hosted by the Financial Times at the World Economic Forum in Davos, Switzerland, two of the biggest…
The Most Complete Repository of Research Links and Educational Content on the VERSES AI Technology & Active Inference AI This…
Computing is changing on every basic and fundamental level. Generative AI is one piece of that computing puzzle. It’s a…
A pivotal roundtable discussion held by the Boston Global Forum, the Active Inference Institute, and the Neuropsychiatry and Society Program…
In an unprecedented move by VERSES AI, today's announcement of a breakthrough revealing a new path to AGI based on…
In a world where AI reigns supreme, there is a question looming among the tech elite: Are we on the…