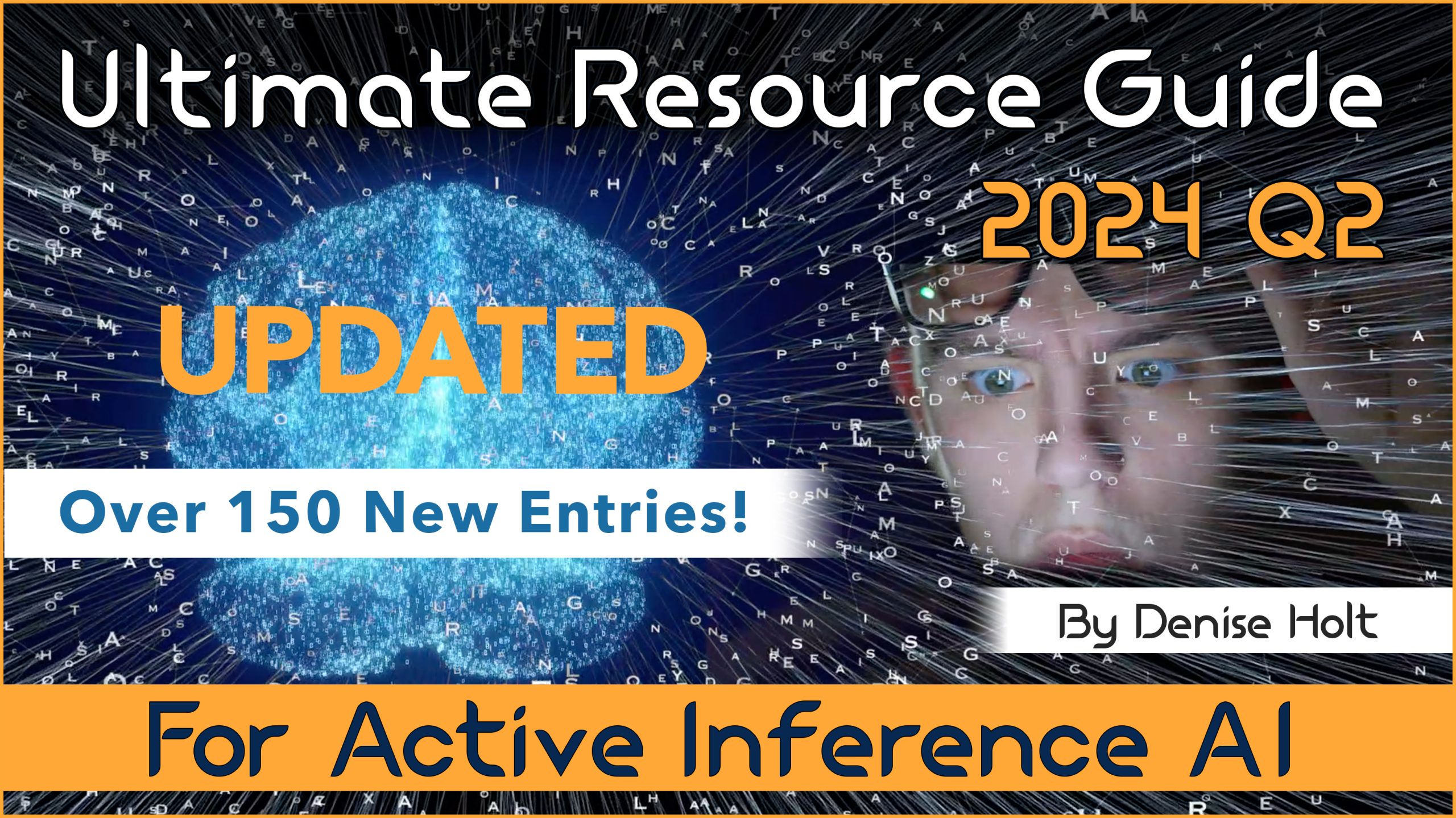
Updated - Over 150 New Entries for 2024 Q2 - Research papers, articles, videos, who to follow, and more! The…
In a recent episode of the Spatial Web AI podcast, Dr. Bert De Vries, a prominent researcher from the Eindhoven University of Technology, shared his insights on the evolving field of artificial intelligence, focusing on active inference agents. These agents are based on Karl Friston’s Free Energy Principle and are set to revolutionize AI technology.
Discover how the Active Inference approach to AI follows the principle of least action and describes how information processing occurs in biological systems like the brain. The brain builds a model of the world to predict sensory inputs and uses Bayesian inference to update its beliefs. Dr. De Vries highlights that this approach creates adaptable and efficient AI systems, with applications ranging from healthcare to robotics. Learn about how this form of AI works in the brain for efficient information processing, applications in robotics, engineering and medicine, advantages over deep learning models, and why active inference is poised to be the next big wave of AI.
Dr. De Vries discussed the concept of first principles AI, a novel approach that derives AI from fundamental assumptions. He highlighted Karl Friston’s free energy principle as a cornerstone of this approach, describing it as a breakthrough in the physics of self-organization and information processing.
The free energy principle, as explained by Dr. De Vries, is integral to understanding the brain’s functioning and has vast implications for AI development. He emphasized its potential in creating AI systems that can adapt and learn in real-time, a significant leap from current AI models like machine learning and deep learning.
One of the key advantages of active inference AI, according to Dr. De Vries, is its ability to learn and adapt in real-time. This is a stark contrast to traditional AI models, which require extensive training and often fail to adapt to new or changing environments.
Dr. De Vries provided examples of how active inference AI could transform everyday devices, such as hearing aids, by allowing them to adapt to changing environments without user intervention. He also discussed broader applications in various engineering fields, showcasing the potential of active inference AI to revolutionize the way we interact with technology.
Dr. Bert De Vries’ insights on active inference AI, rooted in the free energy principle and first principles AI, mark a significant shift in the AI landscape. His discussion underscores the need for a new perspective in AI development, one that aligns more closely with the natural processes of the brain and physics, promising a future where AI seamlessly integrates into our lives.
Visit these links to connect with him: Website: http://biaslab.org, RXinfer Toolbox: http://rxinfer.ml, Company: http://lazydynamics.com
Episode Chapters:
0:00 – Introduction
1:55 – Understanding First Principles AI
8:31 – The Impact of First Principles on Personal Experiences
18:17 – Active Inference and its Applications
25:13 – Advantages over deep learning
26:31 – Building a Toolbox for Active Inference
34:36 – Energy efficiency of Active Inference and the Free Energy Principle
44:33 – Distributed Intelligent Agents
48:41 – Why active inference is next wave of AI
53:33 – Advice for students
58:02 – Wrap-up
All content for Spatial Web AI is independently created by me, Denise Holt.
Empower me to continue producing the content you love, as we expand our shared knowledge together. Become part of this movement, and join my Substack Community for early and behind the scenes access to the most cutting edge AI news and information.
Hi, and welcome back to another episode of the Spatial Web AI podcast. Today I have the pleasure of having a wonderful guest here with us, dr. Bert DeFries, PhD researcher from the Netherlands. Bert, why don’t you tell our audience, introduce yourself, let them know all the amazing projects and work that you’re doing and what field of research are you in?
Speaker 2 – 00:40
Okay, well, thanks a lot for letting me to be on this show. I’ve seen the show and really enjoy the podcast. So my name indeed is Bert DeFries. It sounds Dutch. I am Dutch. I’m a professor at Eindhoven University of Technology, which is a technical university in the south of the Netherlands in the Department of Electrical Engineering. And, yeah, I’m working on active inference agents, which are these AI devices that inherit from Karl Friston’s reality principle. I would love at some point in the conversation to talk about how I came to this field, but I’m very enthusiastic about it. I have a team of about ten PhD students that are working mostly on trying how to realize these agents in software and hardware. So we are not the philosophers of the community.
Speaker 2 – 01:41
We’re the engineers at the bottom trying to implement it and build them and see if we can make great things wonderful.
Speaker 1 – 01:50
Yeah. I’m so excited to talk with you. So why don’t we start? Maybe you can give our audience an explanation of what is first principles AI.
Yeah, well, first principles AI is a term that I saw first, actually, just a few weeks ago in the Hype cycle, gartner’s Hype cycle, and was very excited to read about it because it does describe what we are working on. It’s a new technology and it doesn’t have the hype and the advertisements of generative AI. But this is more powerful. First principle means that this type of AI you can derive from very basic assumptions, and This is what Karl Friston has done. This type of AI follows the principle of least action which is one of the core principles of physics. Karl Friston calls it the free energy principle. And it basically describes a new branch of physics, the physics of self organization, information processing in biological systems, in particular the brain. But it applies much broader than the brain.
Speaker 2 – 03:12
And I think the interesting thing is that in this type of approach, you can derive AI as sort of an emerging property of physics. So I think, looking ahead in 1020 years, that the frail energy principle will be taught as the physics curriculum in high school next to your classical mechanics and electricity, and then you have the frequency principle. So I think it’s extremely big invention, if you can call it an invention, but it’s going to be very big. But it will take a long time because it’s not easy to get your head around it.
Speaker 1 – 04:02
Yeah, so maybe you can help. I’d love to hear your explanation of it because you’ve seen the stuff that I’ve been writing and stuff and I do my best to try to break it down and make it understandable. But I would love to know how you would explain it to somebody.
Yeah. So there is a principle in physics that lies above the laws we know. Newton’s Law and some people know, I remember from high school Boyle’s Law, the Gass Law and there are some other laws that we try to remember. It turns out all of these laws you can derive from a higher, more abstract idea, the principle of least action that says that in nature energy differences are minimized in least time. So if I have an object here, I have here a pen and this pen has potential energy because it’s a gravitational field and it has no kinetic energy. So there’s a difference between these two types of energy and nature will bring this difference to zero. So when I drop it basically the potential energy goes down and the kinetic energy goes up until they meet. And this is how nature works.
Speaker 2 – 05:25
It’s a spontaneous process. This happens also for electrical energy, electrical and magnetic energy differences and other types of differences. And the brain is also a physical organ. So you can ask the question, well, shouldn’t it then also submit to this principle of least action? And indeed it does. And this is the brilliant Karl Friston who described this type of physics in an equation.. So we have an equation of how energy difference in the brain are neutralized. But the interpretation of this equation is that this is how information processing happens. So it describes how your thoughts change and your beliefs when you receive more new information through, let’s say, sensory observations. It describes how your beliefs change, describes perception, but also how perception translates to actions that you make. It describes the learning process, attention mechanism.
Everything that goes on in the brain that relates to information processing can be described as free energy minimization. So it’s a physical process.
The brain doesn’t want to do it. It has to do it because it has to just follow the law of physics. It’s a spontaneous process. Now what it all turns into because that’s just the physics. And so what does it mean? It means that the brain makes a model of the world around us. It tries to predict my sensory inputs for my eyes, for my ears inside the body. It tries to predict my glucose level in my blood, everything. We need to have a model and we need to be able to predict the future of the world. Otherwise I cannot survive if I cross the street.
Speaker 2 – 07:32
I must be able to predict if the car is going to hit me or not.
Speaker 1 – 07:36
Right?
Speaker 2 – 07:37
I’m going to die if I have a poor model of the world, right? But there is a twist. This principle just leads to us building a model of the world. But there is a twist. If it would just be that, then the brain would be a scientist. It would make actions. These are your experiments with the world. You get sensory inputs, you get data, you build your model, and you make new actions, new experiments. You get new data, and you build them all of the world. It would be an optimal scientist because free energy minimization does the right thing from an information processing fuel point. It approaches Bayesian inference, which is what we want to do. If that will be the only thing, the brain will be a scientist. I would predict that this car is going to hit me.
I’m going to walk, and the car is going to hit me. So that would be fantastic. But the brain actually tries to help you to survive. If I roll this model out in the world, and I imagine that this car is going to hit me or in my simulation, then I’m not going to walk. And if I can see that the car is not going to hit me, I am going to walk. So there is a purpose, right? We actually try to predict the future of the world, and we are able to set constraints. We try to predict how we want the world to change. We want the world to change such that I can safely cross the street. That means I stop when the car is close to me, and when the car is far away, I’m going to cross.
Speaker 2 – 09:23
So there is a bias to it. And that means that the brain now is like an engineer, because it’s able to make algorithms to cross the street, algorithms to ride a bike, algorithms to recognize speech, to recognize objects, to learn how to drive a car. Well, that’s great for us, but that’s also great for engineering, because now we have the math of how people are able without any engineers in the loop, because you just go outside and you learn how to ride a bike. You need no engineers. You just go out with trial and error. You learn how to ride a bike. And we know now the math and physics of how that happens.
So in the future, if we are able to harness this math and physics on a small computer, we can put it in a robot and say, go out and learn how to ride a bike. Go out and learn how to drive a car. Go out and do whatever we want, right? Because if I really want, I could probably learn to speak Japanese with enough effort. You can then tell a machine to do that, right? And it’s automated. It’s entirely automated. I’ve been talking too long about it. But this is very exciting for engineering, because the brain is sort of an automated optimal engineering design loop, right? So it’s very worth it for engineering disciplines to try to put this on chips and in computers and use it to automate engineering design processes.
Speaker 1 – 11:14
Yeah. And what’s really interesting to me is that it’s real time, right. So when you talk about the applications for AI, huge difference between active inference AI based on the free energy principle versus the AI that most people are familiar with. Now, the machine learning, deep learning, the LLMs, the generative AI models, and maybe you could talk a little bit about that, about what the advantages are for an AI system that is dependent on these individual intelligence versus the big model, the monolithic approach of yeah, absolutely.
Speaker 2 – 11:59
This is a great question. And this is also why, let’s say current generative AI and deep learning is fantastic, but you cannot take it into the world and tell it to learn in real time.
Speaker 1 – 12:13
Right.
And this is also how I got interested in it because I used to work, I still work a bit for a hearing aid company. And here is what happens with hearing aid. So people buy hearing aid, which is a fantastic wearable, and it’s very complex. There’s a very complex algorithm in there. But you go to your audiologist, they tune the parameters, and then you leave the dispenser very happy. And two weeks later, you sit in a restaurant and you can’t understand your conversation partner because you can’t predict something in the world. Everything. You can’t predict everything in the world. There’s really nothing you can do. Right. You can go back to your audiologist. But this hearing aid algorithm is very complex.
Speaker 1 – 13:05
You mean in a restaurant, there’s a bunch of ambiance noise that’s interrupting the.
Speaker 2 – 13:10
Settings that the hearing yeah, let’s say that will drown out the voice of your conversation partner. And clattering of utensils on the plate is also terrible for people with hearing impairment. So it’s extremely annoying experience in a restaurant if the hearing aid is not optimally set for them. So they go back to the audiologist. This person often cannot solve the problem. It goes back to the company. Then the engineers start working on it for half a year. Then it has to be recoded in an assembly language and then the marketing people get a hold of it. It takes a full year to go around.
Speaker 1 – 13:58
Wow.
Yeah. So, from a complaint by a user until a new algorithm in the field that has been tested and everything right, and has been approved by the FDA, it takes a full year. 20% of hearing aid users so will end up putting their hearing aid in the drawer because they can’t wait a year for their problems to get resolved. So current design cycles for hearing AIDS are not real time. They take a year.
Speaker 1 – 14:28
That’s a long time.
Speaker 2 – 14:31
So what we would want is if you’re in the restaurant and you cannot hear your conversation pine, you just tap your watch. And in the watch is some kind of agent that sends new parameters to your hearing aid and you say, no, that’s not good. That’s not good. Okay, I’ll take it. And then you go on. And maybe you could even do this covertly by wiggling your toe so your conversation partner doesn’t see it. Right, right. But you’re updating your hearing aid in real time and a week later you do another little update and three months later you have a completely new personalized acoustic experience that you have designed, that you have personalized so are you talking.
Speaker 1 – 15:19
About there would be different settings for different environments. Now how would the agents help you to be able to kind of hone in on the proper one rather than.
Having to yeah, so the problem is that these devices have lots of parameters and every parameter can take on lots of values. So the number of parameter values is gazillions. Right. And you can’t go like an hour. You want to do this three times, four times.
Speaker 1 – 15:52
Right.
Speaker 2 – 15:55
And if you put this agent must pick the most interesting alternative from all the possible options. That means if it knows what you like, it should take that. If it doesn’t know what you like, it should try to take a setting that provides the most information about you, that’s information seeking, that has like a 50% chance of you liking it. Because if you then say, I do like it, then it learns something about you. Or if you say, I don’t like it also learns.
Speaker 1 – 16:36
I’m sorry, would the agent then be able to also take in cues from the environment that you’re in, like sounds and different things so that it could go, okay, this is noisier, these noises I’m sensing. So this might be a likely option.
Yes. So if the agent would turn into or the hearing aid would turn into an active inference agent, it would become a device that is going to predict sensory inputs, your inputs, your feedback, and going to say, I’m going to predict that you’re going to be happy. And I will do actions, setting parameters that are necessary to make that come true. Because the agent has to learn what you like, under which conditions.
Speaker 1 – 17:31
Yeah, right.
Speaker 2 – 17:32
And it must do so in least amount of trials because you were likely 75 years old and you don’t want to go every half an hour through a training process. So it turns out that the agent that we want in this watch is really an active inference agent that balances goal driven behavior, giving the right settings to you versus learning about you being proactive. If it knows, oh, you’re in a car now, I’m going to set the parameters that I know that you’re going to like, or if I don’t do anything, you will get feedback whistling. So I’m going to lower it again.
Speaker 2 – 18:17
It should be an intelligent assistant that is not passively triggered by you, but that through interacting with you becomes sort of a seamless assistant that intelligently knows and gives you a great acoustic experience from all the feedback that it has had from you. Right. It should be a learning agent and an active influence agent is optimally suited for that.
That’s fascinating. And when you think of just that as being one use case for how this can just change how a person experiences the know through a device, gosh, what are some other use cases that you see? I mean, I know that when I met you last month, it was in Ghent, Belgium. We were at the active inference, the International Workshop for Active Inference, and you were speaking there, and I believe you were also talking about robotics and different applications in that field. Maybe you could talk a little bit more about other ways that you see this type of AI just really kind of changing the way we live and breathe in the world.
Speaker 2 – 19:43
Yeah. In general, I think active information agents are going to be good at tasks where people are good at and these are usually tasks where current AI systems are not very good at or they have to be trained forever to be good at it. But adaptive behavior is something that we are very good at.
We are very good at adapting when the world changes around us. And in engineering, I hear this almost every day, that people design systems for the hospital medical devices, that it all works fine. It all works fine for exactly the situation that it’s been trained for. And then when the world changes, a bit suddenly breaks apart, right? When you have some, I don’t know, some kind of a heart rate monitor during a surgical operation and the patient starts sweating, suddenly you can’t get a heart rate anymore.
Or somebody opens a window and all the health breaks loose, right? Yeah. And so what would an active influence agent do? It has always this horizon of I need to make the patient happy, or I need to be able if I’m an agent in a vacuum cleaner, I need to clean the floor. Even if the world changes so it will start making explorative actions, like information seeking actions to learn. How has the world changed once I’ve done that, I can fulfill my task again. And you don’t have to tell that to the agent. It’ll do it automatically. This is all baked in this active inference agent, this free energy principle. It’s what we do naturally. When I learn how to ride a bike, in the beginning, since you want to predict the world, all your predictions are off, right?
Speaker 2 – 21:54
So these handlebar minervers are just aimed to elicit data so that your brain can model the world. Once you have a good model, you can predict everything and you can ride the bike. You don’t even have to think about it, right? You can ride anywhere and never think about it’s very much. So when you learn how to drive a car, in the beginning, you can’t even have a conversation with your instructor because you need all the attention on diving.
Speaker 1 – 22:25
It’s like that meme of let me turn down the radio so I can focus better.
But it is literally that right. And you need all your attention because everything you predict is wrong. All your attention goes to updating your model. Once you have a good model of how a car and the world changes as you’re driving and moving, I mean, you can drive for 3 hours, you don’t even know how you got there, right. Because you’re doing all kinds of things, but driving it’s all unconscious, right? But if the world would change, you would suddenly need the attention again. You will learn the model again and know how to drive. Like when you go into another car, when you suddenly get into a truck, okay. Then you need the attention again, right. Because now the world has changed, right.
Speaker 1 – 23:20
The controls are all everywhere that you’re not expecting them to be, all right?
Speaker 2 – 23:26
And this is pervasive in everything, in every device we make, everything engineers make, whether it’s medical apparatus or robots or whatever, the world around this changes. And the robot, if I have a robot and I say you have to go into the building and shut off this valve because there’s a fire, I don’t know if this robot is going to meet sand or concrete inside, right. He just has to explore.
Speaker 1 – 24:01
Yeah.
That’S the future of these agents. And that’s what we are currently in engineering so bad at. We can design great robots for one task. The great example is of course, Boston Dynamics, that meticulously work for weeks on end to make a robot dance in a perfect way that looks stunning. But if you change the world around it’s got to break apart that behavior, because that robot is not programmed to adapt to a changing world. This has been traditionally one of the biggest problems in engineering, not just for robotics and for medical systems and for driving cars and everything, but almost everything we build. People are good at it. Active inference is good at it. Yeah, we seamlessly adapt. This is why active inference could also change engineering, right? I mean, web three, sure, I totally believe in it.
Speaker 2 – 25:13
I completely align with the roadmap of Versus. I think it’s amazing. But it’s broader, it’s bigger than that. It’s also in engineering of intelligent lighting, smart cities, intelligent lighting, even in Eindhoven. Here we have the biggest chip maker machine of the world is ASML. They built the machines that make the chips. They also have lots of sensors and actuators in that thing. And if there is a small change in temperature, then their system, because this is all at nanoscale, their system goes on tilt, right. They have to really adapt. They’re doing a good job, but active inference could really help there as well. So it’s almost everywhere in engineering. The beauty of it is, as an engineer, that there’s only one process, just free energy minimization, nothing else. So you don’t have to worry about machine learning, control signal process.
Speaker 2 – 26:23
You must minimize free energy. Problem is, it’s very difficult to write good code for it.
Well, that brings me to one of the projects, the things that you’re working on. I believe the website is called Rxinfer, but you’re building a toolbox, is that correct? And it’s open source, yes.
Speaker 2 – 26:50
So I just was working on these hearing AIDS forever, because I’ve been 25 years working on hearing AIDS. Eight, nine years ago, I completely by accident read this paper of Karl Friston, a Rough Guide to the Brain, and I didn’t understand it, but I realized this is very special. Right. It took me a long time, took me years to understand what he was really talking about. But I kept thinking, I know it’s important, so it’s worth the effort. And it was. So the last seven, eight years in my research group here at the university, and we focus on building a toolbox for free energy minimization because that’s the only ongoing process. The toolbox indeed is called RX infer. So if you type in, RX means reactive.
Speaker 2 – 27:48
It also means a prescription and drugs, but it means in computer language, it means that it’s a spontaneous process, if you will.
Speaker 1 – 28:00
Okay.
Speaker 2 – 28:03
What does it do? It minimizes free entity, this toolbox. And so it is hopefully going to be the engine for active inference for other companies that want to develop active inference agents. Right. The unity for games or the TensorFlow for machine learning. We try to build a toolbox like it to power active inference agents.
Speaker 1 – 28:32
So how does it work? How does that toolbox work? Because I know that a part of my audience recently, especially with what came to light with the research team at RIKEN in Japan proving that the free energy principle is indeed how neurons learn. And I’ve written a couple of things and know also talked about what the Versus white paper is and what they are building and doing. And Carl is working directly with Versus as their chief scientist. So now it’s like I’ve had people from the open source community that they’re really paying attention to these things. What would you tell them about this toolbox and what kind of lead them into where they should be focused and headed? And how can they get started?
Speaker 2 – 29:28
How can they okay, I’d like to say two things about I want to say first, why, if it all would be working, why it would be quite a revolutionary thing for engineering. I don’t mean our specific toolbox, right. But in general, if these toolboxes were available, right? So currently, if I write an algorithm for a robot to navigate, it would take 40 pages of code because it’s just a very complex algorithm, and lots of engineers would be working on it, and half these engineers would probably leave the team after three years for
better options. And so if your company and you build a robot and you have a code in there, then after three, four years, almost nobody in the company knows the code anymore. So it’s a real problem.
If this is going to work, this active inference, then the only ongoing process is active inference. Active inference is a process in a generative model, in a model of the world. But to just write the model down in code is less than one page, almost always. I mean, the most complex model is not going to be more than one page. So in the future, I’m talking 510 years, if you would build a robot and there would be good active inference or free energy minimizing toolboxes, this robot company would write one page of a model, and the other 40 pages are still there. But this is the toolbox that you just get a license for. This is just French minimization, which is nothing but a formalization of proper reasoning with information, just like the brain does. It has nothing to do with robotics.
Speaker 2 – 31:26
It has nothing to do with hearing AIDS. It’s just proper reasoning. Right. And if we can automate it in the right model, then you can write a complete hearing aid algorithm in one page of code plus inference, and a robot in one page of code plus inference. So it becomes much better, maintainable less errors. The companies don’t need 50 60 engineers just to maintain a piece of code. So it would be very important. Now, we started like seven, eight years ago with this idea we want to build a toolbox because active inference is fantastic. But if I want to make a synthetic active inference agent in the world that works, it has to minimize free energy, and it’s incredibly hard to do it. To derive the equations manually, it’s almost impossible. I cannot do it. Right, right. Yeah.
So for a nontrivial model, it’s not possible to derive the equation, so we have to automate it in a toolbox. So we worked on that. And the interesting thing about this toolbox, RX infer, is that now we are an engineering group. I don’t care about biological plausibility, really. If it works. But if you look at it so how does it work? It works by we have a model that is a graph. There’s nodes in these graph, they are connected. They send messages to each other. And this message sending is a spontaneous process that minimizes free energy. Whenever there’s an energy difference between two nodes, they send a message. If you look at that, you think, well, that’s like neurons that are connected with axons.
They send action potentials. It looks the same way.
Speaker 2 – 33:19
So while we didn’t set out to be biologically plausible, it turns out how biology solves the problem is the best way to solve it. I mean, we end up with a toolbox that looks very biologically plausible. So there is a reason why the brain is a network of nodes that send messages. There is a reason because that’s the way to solve it.
Speaker 1 – 33:48
You bring up a really good point too, because one of the things to me with the machine learning, deep learning models is that the energy draw just to operate them doesn’t seem very sustainable to me. But when you’re talking about how biological, how the biological reasoning works and how the brain is so efficient with energy, maybe you would be able to explain that a lot better than me as far as why this is such a scalable and energy efficient direction to go in for AI.
Yeah. Our model consists of a big graph with nodes. And these nodes just decide by themselves if they’re going to send a message to another node. It’s a spontaneous process and this is the whole process of free energy minimization. Now, if I have a neural net, a regular deep neural net, there’s also nodes that compute messages and send them to other nodes. So you could say, okay, well, you’re building a neural net, but there’s a difference. And that when you do free energy minimization, you have a probabilistic model and you’re doing Bayesian inference that means, yes, we predict a world, but we also predict confidence around it. If I am driving and I see an approaching car, I am predicting the path of that car and I’m going to spend my computational resources on avoiding to hit that car.
Speaker 1 – 35:41
Right.
Speaker 2 – 35:42
I can do that by trying to predict the path of the oncoming car in quite an accurate way. I also predict, and you can think of free energy as the prediction error divided by the width of your arrow bounds.
Speaker 1 – 36:06
Okay.
So if I try to predict very accurately the path of the car, I’m going to have a small width and I’m going to divide a small thing. That means if there’s a small error, this term blows up and I am going to spend all my resources on minimizing that. I will also predict that the car is blue and turns out to be red. But I predicted with an extremely large arrow bounds, which means that if the car has another color, I’m going to divide it by such a large term that the free energy difference is nothing and I don’t pay attention for it. This is a mechanism.
Speaker 2 – 36:51
I’m not sure if I explained it well, but this mechanism makes it possible for us to look everywhere in the world to hear, to have a tremendous amount of information flowing in the brain and only spend our resources on a very small part, on the part that we need that’s most, let’s say, pressing to survive an oncoming car. Walking with running with a scissors.
Speaker 1 – 37:21
Right. I totally get what you’re saying. Your brain is not going to be trying to figure out the puzzle when you’re focused on the problem at hand.
Yeah, because in my. Model or my model, let’s say, in arcs infer, if you divide by big arrow bounds, the process stops and it will only process that car. Yeah, it sees it’s a red car. It doesn’t care. It doesn’t do anything with it. In a neural net, there is no confidence bounds. You have to process everything. You have to put all your resources on the path and the color, and you cannot adaptively change what you pay attention to. You spend your resources on and whatnot, suddenly there may be a bicyclist on the right hand side. Now I have to also spend resources on that and I get a little bit less resources for the car because we have only 20 watts to cope with in the brain.
Speaker 2 – 38:25
So we are constantly dividing our attention to various things that a regular neural net can never do. You can only do it when you embrace the Bayesian framework that well, basically that Tristan has described. You can’t do it with a deep neural net. You can’t do it with a non probabilistic generative AI. So that allows the brain to process huge amounts of data and still basically consume the power of a small light bulb. Right. 20 watts is nothing. It can’t even light up your room, right?
Speaker 1 – 39:06
Yeah. No, that is so interesting. So then let me ask you this, because if regular neural nets, right, if they don’t have that ability to kind of hone in their attention where it needs to be at a given moment, right? Then they’re in this state of continual wide net multitasking right, and trying to predict whatever it is, the next token of what the task is. Right. But then you have the multimodal models where they can then kind of like do one task and then pass it on to the next one that’s skilled at this task and then that kind of thing. But with the active inference and the free energy principle, then that’s the advantage of multiple agents. Right.
Because then multiple agents can use this way of inference that’s just from their own frame of reference and their own view of their model of whatever the world is, from whatever they’re coming. But then they can also learn from each other at the same time. Correct. And that’s how you can have this huge network. But it’s still extremely energy efficient. Am I thinking about that correctly?
Speaker 2 – 40:31
Yes, absolutely. Let’s say the Bayesian framework, because the entire process deals with uncertainties and not spending attention, not spending computational resources on stuff that’s not important, that has a lot of uncertainties, but is not important. So it processes it. But in the background, right? I can look into my room and I see the chairs, but I’m really not spending resources on it. Right. I’m spending resources on that when I suddenly see a lion there. Right, right. So that’s certainly how these agents work. Having this network intelligent intelligence makes it also far more robust. And that’s a bit like when you make things modular and you try to sort of keep all the information processing in a module and have very spontaneous communication between modules, then here’s the difference.
Speaker 2 – 41:46
So if I would write a fixed algorithm on how to avoid this car, I would say, okay, I do this and then try to get that path. Then I’m going out with my algorithm and he’s doing his job and suddenly there’s a cyclist right next to me. Stuff happens in the world, right? Unexpected things. Well, damn my algorithm. I didn’t expect there to be a cyclist I can’t adapt. So the thing is, in the real world, your algorithms have to be able to spontaneously adapt. One of the things that it has to have to adapt to is if I burn out, some neurons in my brain has robust to death, right?
Right.
Speaker 2 – 42:37
Some neurons may die. If I take a computer code over here, do this, do this, and I randomly burn out two lines, this computer code will crash and burn. But if I take out a couple of neurons out of my brain, I’m still functioning, right? And this is a bit like you should think of it as throw water off a mountain, right? It zigzags its way across the rocks and whatever. Then you put in a new obstruction with some shelf and it just goes around it. It just finds a new path. You put up an obstruction, but the world didn’t know that. It just spontaneously finds a new path. It spontaneously adapts. And this is the spontaneous free energy minimization process. That’s how AI should work if the world changes, but it doesn’t if you want to program everything beforehand.
Speaker 1 – 43:45
Right? It’s interesting because to me, I think of stroke victims, right? But the brain just automatically starts to relearn and somebody who has had a major stroke, I mean, it’s interesting to me because when they start to relearn and even motor skills, if that’s been affected and stuff like that, a lot of times people say, wow, it seems like a completely different person.
Which makes us realize how fragile our brains are for who we become, all based on this learning process, learning of our environment. But the brain does spontaneously just do it on its own. These stroke victims will just automatically start to relearn and remodel the world.
Speaker 2 – 44:33
Yeah. It’s like putting up an obstruction and again, it just finds a new path around the obstruction, right?
That wouldn’t happen if everything was pre programmed.
Speaker 1 – 44:46
It would break.
Speaker 2 – 44:49
Yeah. So what RX infer tries to implement that by not specifying beforehand which node is going to send a message to which node, because, hey, this node may not exist in a year from now, right? Every node just tries to spontaneously find out, is there an opportunity to minimize free energy if I send out some messages the next node that receives messages says, well, is there an opportunity to minimize free energy? So I send out messages, and if that node gets burned out because some transistor burns out, then there is other nodes around it that take over its task. So it’s a very soft landing if something happens. And this is when you talk about distributed intelligence, distributed AI agents everywhere, small agents that do their task.
influence agents, other agents will learn to take over the task, not because they want to. It’s just how frequent minimization works. It’s just how the physics works. It’s just how it’s set up. And so that’s, I think, very exciting. It will make AI systems more robust. It will conserve energy because you’re not going to spend energy on stuff that you don’t need to accomplish your task. Yeah. So there’s a huge future for this, but it’s a difficult future in the sense that it’s not a simple trick to learn. Right. It’s really hard from I mean, we’ve been working on it seven years. We’re not the smartest people in the world, but we’re not the dumbest either. No, it’s just a difficult thing.
Speaker 2 – 46:56
And that means that it’s going to be a slow moving of an oil tanker towards this direction.
Speaker 1 – 47:07
It’s fascinating to me. I was going to ask you, why do you feel active inference is the next big wave of AI? But I think we’ve really covered that. I think it’s kind of a no brainer because like you said, AI, especially if we’re talking about AI that’s going to be running mission critical operations or like hospitals or airports or things that have to be able to predict and adjust in real time, even just taking autonomous vehicles, like autonomous cars. It’s funny because I’ve seen Gabriel Renee, CEO of Versus, he’s described a couple of times in some of his talks that you can train a machine learning AI to recognize what a stop sign is.
But if you put that stop sign in the back of a moving truck, it’s not going to know what’s going on because it’s going to think it should stop. It doesn’t have the context and the ability to just adjust and perceive what’s going on in real time. So I think that’s such an important thing for people to understand when we’re talking about AI that needs to do real critical things like operating.
Speaker 2 – 48:41
I think that’s a great example. Also, I really love the vision of the VERSES team, right. Our role. We try to be an engine in that vision. Right. We are not the philosophers, but just try to be an engine in that. But yeah, he’s entirely right. But the example that he gives also speaks to the same idea that if the world changes around you, then you want to have an AI that recognizes that updates its model in the most efficient way and continues its operation. Right. And it should be smooth. It shouldn’t have to think about it. It just happens automatically. This is a problem for engineering because we tend to design our algorithm at a desktop and then we put it in the world and there is very little learning in the world.
Speaker 1 – 49:45
Right.
And active inference is totally the opposite. Right. It’s completely in the world. And it works because well, because it works just like the physics, basically, and it has a cost function and you can talk for hours about how beautiful all the properties of the free energy if you decompose it into different terms. You can talk about it tries to build the simplest model or it tries to build, let’s say, just a good enough model and just a good enough solution. It doesn’t spend any computation on building more precise models than are needed because you need to save your energy. So everything works out there beautifully. It’s just a matter of growing the community. I mean, 30 years ago I’m really old, but those 30 years ago I actually went to what’s now called the NeurIPS Conference, which is the biggest machine learning conference then.
Speaker 2 – 50:53
It was called Nips and it was in Boulder in Colorado. This was in 1990, I was a PhD student and I went there and there were 60 people. 60 people were presenting and I think five years ago there were 6000. And just because they limited, right, maybe it’s like 10,000 now, because if they would open it up, there would be 50,000 people going to the conference. There were 60 people. And that’s about the amount of people that were at EY. So I think it will go faster than 30 years for active inference. Yeah, but it’s a process that even if it doubles from 80 to 160 to 320, it’s still slow in the beginning before it actually takes off. Right?
Speaker 1 – 51:42
Yeah. Right. Yeah. When you talk about the law of accelerating returns and things like that, it always seems slower in the beginning, but it’s really moving at lightning speed when you talk about the gain over time. But, yeah, I totally understand what you’re saying. So let me ask you then, if you were to speak to students who are wanting to get into the field of AI that are wanting to start thinking about how to work towards this future and what they should be doing, what would be your advice to them?
Well, of course it depends a bit on which field the students are, if they’re psychology. But for engineering students, I think one of the great ways to start is try to read one or two papers. Simple papers by Kalfish and A Rough Guide to the Brain is an interesting paper. You will probably not understand it and then listen to podcasts like your saw. There’s a nice podcast with Kyle Frieston, with Lex Friedman, right? And also Tim Scarf and Keith Dugger have a machine learning street talk podcast. So listen to the podcasts, there’s lectures online. And try to think about it. Try to think about the weaknesses of the systems that you see around you.
Speaker 2 – 53:33
The thing is that in the machine learning community, the top people in the machine learning community, they say nowadays we don’t need to look at the brain because machine learning is going to go way past the brain, right? So they’re not really inspired by the brain anymore. But the brain solves tasks easily that our current AI cannot solve, like riding a bike. And it does so with a million times less power consumption than a regular computer. So for me, that’s hugely inspiring, right? It also does it with exactly the same brain. It’s the same brain that can learn Japanese, ride a bike, drive a car. It’s the same brain. So how is it possible that with one brain you can basically solve all these problems that you want to solve?
Speaker 2 – 54:34
It’s an amazing feature that with the same brain you can solve all these tasks just by going out and by trial and error, just by doing it. You don’t need 300 people in the loop, engineers and whatever. It’s completely self organized, low power, low latency. It has everything that AI currently lacks. Current AI also has some beautiful properties, but this is far more powerful. So I think it’s hugely inspiring. For students, it’s difficult, so you have to get through some you have to be a bit persistent, right? But I think it’s totally worth it, right?
Do you think these agents will end up becoming so self organized and autonomous that they end up doing the programming?
Speaker 2 – 55:36
I think eventually you want to describe these agents just by describing desired behavior, and then the program for these agents will be written. So let’s say if I want to design an agent for a cleaning robot now I have to write a huge algorithm, right? But what I would want to do is describe,
say, okay, you are a cleaning robot, which means you have to apply suction to the floor until the floor is clean, don’t touch any objects, and when you’re done, return to the dock. So that’s desired behavior. And I think from that point, there should be a computer program that says, okay, that means I need to have a camera. I need to have an appliance that applies suction. I need that camera. I need to have object recognition. I can’t touch objects. I need to remember where the dock is.
Speaker 2 – 56:47
So I need to build a model and then automate free energy minimization. So hopefully over many years, ten years, an engineer will just need to write desired behavior. And that’s just half a page of code. And then indeed, this agent, you don’t have to say what the room is like. It will figure it out, right? I mean, you don’t need to tell them how to get back to the doc if it cannot find it’s going to figure it out because it’s going to generate prediction errors until it’s found the doc. So it’s going to keep trying it in the base, optimal way, in the most information, let’s say efficient way to find the doc. It’s just going to do its job. It’s going to take a lot of work to get to that stage. But that could be a future.
It’s hard to predict whether it’s ten years or 15 years, but that could be the future. Right. That you just tell an agent what it needs to do, and it will do it. Right.
Speaker 1 – 57:54
Very cool.
Speaker 2 – 57:55
Yeah.
Speaker 1 – 58:02
To kind of wrap up our conversation, here a couple of things. One, is there anything that we’ve missed? Anything that you feel is important, that we should be kind of letting people know about this field of AI?
Speaker 2 – 58:20
I think we’ve covered a lot of ground, and I felt I’ve said the most important things, and it’s almost like I talked very fast and because I’m enthusiastic about it. Right. So I think I’ve said most of the things I wanted to say. Probably later on this evening, I’m going to think I would have wanted to talk about that.
Speaker 1 – 58:46
Well, I would love to have you back anytime.
Okay. But I think that I’ve said what I wanted to say. It’s just a very exciting field as an engineer to be in this field, because I’ve seen, and I still see every day around me, the complications of engineering difficult systems. And this feels like you’re like designing at a higher level. You don’t do the engineering. You just think about desired behavior and try to automate the whole engineering process. Active inference is an automated design cycle, so it feels almost like thinking at a higher level. It’s very satisfying as an engineer to work in this field. It’s great community, to the point even. And maybe you’ve had that as well, because this relates to how the brain works. Right.
Speaker 2 – 59:45
So when you have issues in your life, even psychological issues, I kind of can explain it now better because I have a bit of better understanding of how my brain works. Right, right. I think it’s not only professionally for engineering, but just general for your life. It’s just good to be interested in this field because it pays off if you understand how the brain works.
Speaker 1 – 01:00:21
Can I ask you a question? And this might be steering it in maybe not even an accurate way, but in thinking about it, like what you’re saying since I’ve been gaining an understanding of the free energy principle and how our brain works when we’re thinking and making these predictions about our life. And when you think of the other research that’s been done in the realm of physics as far as how powerful our thoughts are and as far as to direct reality. And then you think of physics on the level of entanglement and superposition and things like that.
So I know our brains work in this way of minimizing the variational free energy and it’s for survival, for being able to actually interpret the world around us correctly so that we can operate within the world and function in a way that is beneficial to us and our existence. But also, and I think that’s why a lot of people, they’re uncomfortable with change or really uncomfortable with the unknown because it’s deep in our brains to want to be able to know what’s happening. It’s survival. But then there’s also people, and I feel like I’m one of them, where I kind of like the surprise. I like having things in a state of not knowing because to me that’s where superposition lies, right? It doesn’t fall into place until it’s observed.
Right?
Speaker 1 – 01:02:07
So when everything is in this state of kind of superposition, then all possibilities are in play. Right? So how does that work into this? Because to me that’s kind of a fascinating thought and I don’t even know if that’s correct to apply it in this sense, but it does make me wonder.
Speaker 2 – 01:02:32
In the free energy principle and it’s just how the equations work out, right? There is room for curiosity. There’s value to exploring the unknown. There’s value in that because there’s information to be gained. So for you like it when there is a lot unknown because there’s a lot of value to be explored there. In the French principle, that is valued. I mean, that means you actually move to explore that space because you can uncover a lot of information there. So I think it makes sense. I’m not Calfrist, so for the authority you have to ask somebody else. But with my type, the way I think about it makes sense. There is an explanation for the fact that people are curious that they like unknown spaces because there’s a lot of information to learn there. Right.
Information seeking behavior is really part of what minimization of free energy does. It tries to uncover and find information because you may actually find very useful information in those places. Right?
Speaker 1 – 01:03:57
Right.
Speaker 2 – 01:04:00
I think that totally makes sense. And this is a bit of a difference from other frameworks that are non probabilistic to get back to crossing the street.
So you have this model, you run it forward and if the car hits you in your rolling forward in your simulation, then you’re going to stop. If the car doesn’t hit you’re going to walk. But there’s the third possibility that you think it’s too close to call, I’m going to take another look, I want more information. The fact that this third possibility means that there’s value in you saying I need more information, there’s still uncertainty. So that has in that particular instant more value than the options, walking or staying.
Speaker 1 – 01:05:02
Right?
Exploring the unknown at that moment is the most valuable option. You can only do that if your framework is if your cost function, your French is a function of beliefs over states of the world. If I would fully know the world, I would never have this uncertainty. I would never feel like, oh, I need to explore because I know everything. Right. So the cost function to minimize and this is just paraphrasing Calfresten, is a function of probabilities of beliefs of states of the world. And in most engineering fields that try to do similar things, reinforcement learning, control theory, they use another cost function. They don’t use a function of beliefs of states, but just a function, a value function of states. And they would miss out on the value of exploring the unknown. Right.
Speaker 2 – 01:06:08
So they would say, okay, I’m going to walk or I’m going to stay, when actually they may not have enough information. They actually should say, I’m going to stop, look again, wait a little, get more information, and then make my decision interesting information.
Speaker 1 – 01:06:24
So we are making actions that are helping us to keep it in that space to gather more information, make better decisions.
Yeah, we’re always balancing what’s the best thing to do, right. Going for the goal or getting more information. Right. And balancing that.
Sometimes it’s 60% this, 40% that, and it’s completely seamless. Right. So we all have this experience when you say, I need to take a better look.
Right? Yeah. And that saves us, really. But this can be you can think of this as a much broader context outside of traffic, just the fact that you value spaces where there is a lot of unknowns, a future that is unknown, and you love to explore it. Right, because there’s value to be.
Speaker 1 – 01:07:19
Absolutely, yeah. Well, okay, so, Bert, how can people reach out to you directly? How can they find out more about the work that you’re involved in and that you’re doing and your research? Where should people be looking to get in touch with you or to follow your developments?
Speaker 2 – 01:07:40
Okay, so there are three websites. There’s a website of my lab. My lab at the university is called Biaslab, bias Lab. And there you find all the research we do, all the PhD students. I’m also there. There’s the toolbox RX infer. If you just type in RX infer, it’s going to be a ML for machine learning and Malta. And then we have a startup company called Lazy Dynamics that tries to basically find clients that want to work with us exploring active inference with ARX infer. Right. So you could also then go to the website Lazydynamics.com. So I think one of these three websites, you’re going to find an email address and everybody who wants to can just write me an email and I’d love to talk to anybody who’s interested.
Awesome. Well, thank you so much for being here. And I will put all of those links in the show notes with the video. And it has been such a pleasure having you here on the show with us today. Dr. Bert Debries. Thank you so much for being here. This has been really fun for me.
Speaker 2 – 01:09:05
Well, same here. I really enjoyed it. It was really nice talking with you.
Speaker 1 – 01:09:10
Yeah. And I would love to have you back anytime.
Speaker 2 – 01:09:13
Sure. I would love to. So let’s do that. Yeah.
Speaker 1 – 01:09:16
Thank you so much. We’ll talk to you soon.
Speaker 2 – 01:09:19
Okay. Bye bye, then.
Speaker 1 – 01:09:20
Bye bye.
Updated - Over 150 New Entries for 2024 Q2 - Research papers, articles, videos, who to follow, and more! The…
VERSES AI has unveiled a groundbreaking framework that "sets the stage" for ASI, and could have profound implications for the…
In a panel hosted by the Financial Times at the World Economic Forum in Davos, Switzerland, two of the biggest…
The Most Complete Repository of Research Links and Educational Content on the VERSES AI Technology & Active Inference AI This…
Computing is changing on every basic and fundamental level. Generative AI is one piece of that computing puzzle. It’s a…
A pivotal roundtable discussion held by the Boston Global Forum, the Active Inference Institute, and the Neuropsychiatry and Society Program…
In an unprecedented move by VERSES AI, today's announcement of a breakthrough revealing a new path to AGI based on…
In a world where AI reigns supreme, there is a question looming among the tech elite: Are we on the…
"What you're about to see is akin to, I believe, a moment like the launch of the first rocket or…