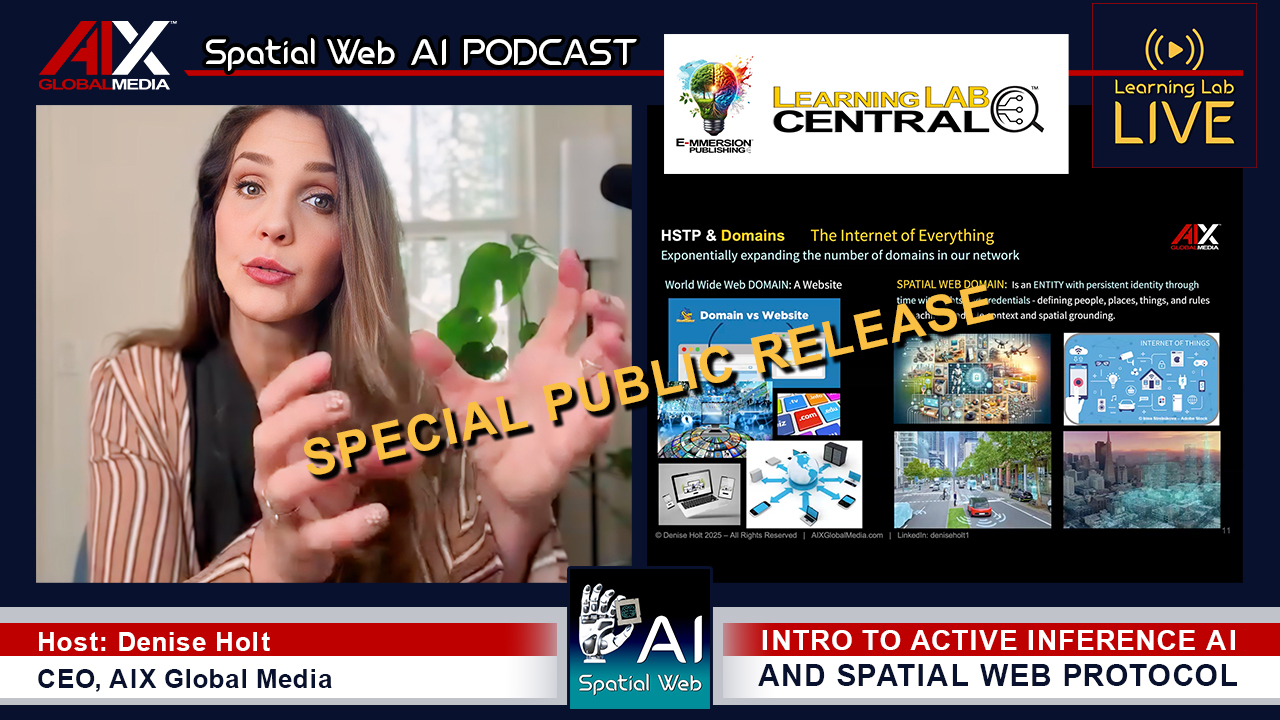
The convergence of two groundbreaking technologies is reshaping how we think about AI, automation, and intelligent systems, affecting every industry...
In one of the most intriguing episodes of the Spatial Web AI podcast that I’ve had the privilege to host, I recently sat down with Daniel Friedman, co-founder and president of the Active Inference Institute. The insights from this conversation promise to shine a light on the curious realm of active inference and its potential to reshape our understanding of decision-making processes and the future of AI.
For the uninitiated, active inference is not just another fleeting term in the vast landscape of technology. As Daniel explained, it embodies a unique approach that merges perception, sense-making, decision-making, and action. Its wide-ranging application, from cybernetics to signal processing, showcases its versatility.
Central to this concept is the free energy principle, which underscores the idea of minimal action in informational systems. To simplify, think of active inference as a method to reduce surprises in predictions by enhancing perception. This capacity to refine awareness, particularly in response to new stimuli, is a game-changer for AI, offering it the capability to decipher real-time environments through sensory input.
One of the pressing questions in today’s AI landscape is the quest for model interpretability and explainability . Active inference is garnering attention for precisely this reason. It offers a model that provides both interpretability and explainability. More so, it emphasizes the importance of actions within dynamic systems, setting the stage for AI systems that interact more naturally with their environments.
Daniel also touched upon the significance of active inference in developing generative models, underscoring its profound implications in the future of AI modeling.
A notable highlight from the interview was the mention of Dr. Karl Friston’s role as Chief Scientist with VERSES AI. Recognizing the potential of the Spatial Web Protocol to translate Active Inference theory into tangible applications marks a significant leap in the realm of AI.
Another intriguing concept broached during the conversation was that of Markov blankets. These play a pivotal role in active inference and the free energy principle, delineating the statistical interdependencies between nodes.
“Enacting Ecosystems of Shared Intelligence”, the upcoming Applied Active Inference Symposium scheduled for August 22, 2023, isn’t just an event; it’s an invitation to explore the practical applications of active inference. With last year’s focus on robotics and its intersection with active inference, this year promises to delve into how active inference can be applied more broadly, especially in online science and participatory ecosystems.
Daniel’s enthusiasm for the symposium was evident. He highlighted the potential of online science and the participatory ecosystems they’re fostering. For those who are curious and wish to immerse themselves in this realm, Daniel pointed them towards activeinference.org. Whether you’re looking to join learning groups, access courses on active inference, or simply expand your knowledge base, this platform is a treasure trove of resources.
This latest episode of the Spatial Web AI Podcast, with Daniel Friedman, is not just an informative session; it is a clarion call for researchers, scientists, and enthusiasts to delve deeper into the world of active inference. With its potential only beginning to be realized, Active Inference is poised to redefine the foundations of AI in the years to come.
Curious to learn more about Active Inference? Visit the Active Inference Institute.
Be sure to register for the 2023 Applied Active Inference Symposium to hear from some of the most brilliant minds in the space like VERSES AI Chief Scientist – Dr. Karl J Friston, Director of Research – Maxwell J D Ramstead, Research Scientist – Mahault Albarracin, and many more.
As mentioned in the interview, here is where you can find the VERSES AI whitepaper titled, “Designing Ecosystems of Intelligence from First Principles.”
#activeinferenceai #FreeEnergyPrinciple #KarlFriston #spatialweb #AIEducation
All content for Spatial Web AI is independently created by me, Denise Holt.
Empower me to continue producing the content you love, as we expand our shared knowledge together. Become part of this movement, and join my Substack Community for early and behind the scenes access to the most cutting edge AI news and information.
00:12
Speaker 1
Hi everyone, and thank you for tuning in to the Spatial Web AI podcast. My name is Denise Holt and I am your host. And today I have the great pleasure of welcoming Daniel Friedman to our show. Daniel is the co founder and president of the Active Inference Institute. And welcome, Daniel. It’s great to have you on our show.
00:36
Speaker 2
Thank you. Glad to be yeah.
00:39
Speaker 1
So maybe you can tell our viewers a little bit about know and what is the, you know, what is your mission or purpose.
00:52
Speaker 2
All right, great. Well, personally, I’m a postdoctoral researcher in Davis, California, so I completed my PhD in 2019 studying ant behavior and genetics, and during that time was getting really interested in ways of thinking about sense making and perception one. Hand and action and decision making. On the other hand, and was learning about active inference and the free energy principle, which I’m sure we’ll talk a lot more about. And since 2021, with a bunch of other colleagues and volunteers and all kinds of contributors, we’ve been collaborating around the Active Inference Institute, which is an open science institute that’s supporting all different kinds of projects and infrastructure, helping people do learning and research in and around active inference. So I’ll just leave it at that for now.
01:48
Speaker 1
Okay, sure. So my viewers, they hear the term active inference from me a lot, and it’s in relation to the active inference AI. That’s part of the ecosystem that’s being created by versus AI within the spatial web. But I think that it would be helpful if they had a much clearer understanding of kind of what is active inference, what is the free energy principle, what are the basic mechanics within that methodology?
02:23
Speaker 2
Awesome. Well, it’s a great question. I think it’s a question that beginners and those with a lot of familiarity continue to ask themselves. So I hope that even if you’re just curious about this and hearing about it for the first time, that you keep wondering about what active inference is. Because truly many of the researchers are still pushing the frontier and it’s really early days overall, but of course I can just give a few definitions or a few angles that might provide a few on ramps. On ramps, plural, because there’s definitely no single way. You mentioned that a lot of your audience is going to be familiar with machine learning. So there may be a way to take what one has learned in machine learning and then connect it with a few differences to active inference.
03:08
Speaker 2
On the other hand, there’s a huge number of people in the ecosystem that have backgrounds in communication organization. All these different areas and a lot of different specific domains like logistics and robotics, where even if the person’s not working hands on with Bayesian statistics or a programming language or something like that. There’s still a lot of embodied and kind of cool on ramps as well. Broadly, though active inference is an approach to modeling systems that have input output relationships and that is one hand very general, that models passive systems like a rock and it can also model very active systems. And it’s those developments in modeling active systems that has been picked up and focused on by fields like cybernetics and signal processing, sense making and then on the action side, more of the decision making and action selection.
04:14
Speaker 2
So active inference is broadly in the lineage of these other approaches towards modeling active systems and there’s a few new connections and developments that make it especially exciting. But just at a first pass, it’s an integrative way to model a continuum of systems from simple inert systems on through these complex ecosystems of interacting systems. And that gives the expressivity to describe the kinds of cyberphysical and high reliability systems that we might be interested in, for example, if we’re using AI in real systems.
04:50
Speaker 1
Yeah. So then what is the free energy principle? What does it have to do with active inference?
04:58
Speaker 2
So, very good and deep question, truly. So I’ll just give a first thought. There’s a category of principles known as principles of least action. And so it sounds initially like it’s going to be kind of like the laziest or the least active path, but actually the path of least action is kind of just like the most likely thing to happen. So if a baseball, given the initial conditions, given the direction that the baseball is heading, it follows a parabola and we call that kind of motion like classical, it’s not exhibiting any quantum effects. We’ll just put those aside for now like large objects follow that kind of smooth, predictable path of least action. And the free energy principle, again, bringing many different fields together information sciences and thermodynamics and a few other areas provides a principle of least action for informational systems.
05:58
Speaker 2
So broadly, for the kinds of information landscapes that are described by Bayesian statistics, it’s kind of like a ball rolling downhill or a baseball following a parabola, some analogy to that in an informational space based around how incoming information update the way that things are in a model that’s being carried forward through time.
06:21
Speaker 1
Okay, so then how does it work from my understanding of it, the free energy principle and active inference, you’re refining your perception so that you can act, you’re acting on your environment to increase the refinement of your perception so that it minimizes surprise, it minimizes uncertainty. Maybe you could go a little bit into those aspects of it and how that makes for better decision making.
07:00
Speaker 2
Great questions. So it’s all there in the title with active inference. It’s bringing together in a kind of integrated composite or synthetic model processes that we associate with perception and sense making one hand and cognitive processes like memory and attention and then also the action component of a system and they’re all being considered in this integrated way. So coming more from the free energy principle, the relationships amongst perception, cognition, action and then impact in the external niche, those are defined a lot more analytically, which is to say in terms of equations. So that is kind of like theoretical physics free energy principle side. And those equations have a lot of very interesting relationships that are very deep to learn about and they also don’t provide any specific implementation order, so they don’t provide a specific pseudocode.
08:07
Speaker 2
So where you get towards the implementation more like the how of it really playing out. It’s in the source code of the kinds of industrial systems as well as research papers and open source artifacts where we actually see like Python or Julia or other programming languages that are being used to implement active inference. And so active inference is a specific approach towards modeling that perception action system you mentioned minimization or bounding of surprise. So when we’re thinking about an active inference agent, one of the key terms which is a quantity that it reduces is the variational free energy or VFE. And the VFE is like a tractable optimizable heuristic that bounds surprise. So the better and better you’re doing on VFE, the closer you’re getting to minimizing your surprise. Minimizing your surprise is equivalent to maximizing model evidence.
09:08
Speaker 2
If you knew exactly how surprised to be by every piece of incoming information, that would be equivalent to saying that you had the best possible concept or situational awareness because you’d be minimally surprised by what was coming in. So model evidence is maximized, which could be for perception or could be for inference on action we can get to. But the model is being optimized in real time and that’s the variational free energy which is juxtaposing incoming data with beliefs about the world. And that’s kind of like the surprise bounding keep me alive, keep me in the flow. And then the second quantity which is related to action selection is expected free energy.
09:54
Speaker 2
But the active inference agent, two of the key quantities that play a role in the sense making and the decision making are the variational free energy, which bounds surprise, and the expected free energy, which does something very similar but for prospective states that haven’t happened yet because it’s kind of like decision making and planning, right?
10:14
Speaker 1
So with this feedback loop, then you’re manifesting new awareness, right, with each cycle of it that helps to increase your understanding of the environment, of what to expect. Would that be an accurate way of describing it?
10:36
Speaker 2
I might say that active inference gives us the grammar or the expressivity to design systems that might have that kind of growth of awareness with new stimuli as surely human and other systems do. And the reason why is because active inference, it’s kind of like a Lego kit or like a toolbox or a set of approaches or modeling tradition because you could make something that’s very educational. So it’s very simple and it’s just based on a two x two world and an agent that moves back and forth or up and down. Or you could develop a model of a specific biological system, or you could research kind of theoretical properties of different models. Some of those.
11:25
Speaker 2
I guess the interesting question will be is how can we develop augmented systems, whether it’s more on the human in the loop side or more on the autonomous side that do support learning from new data, and especially how to develop these kind of higher cognitive and metacognitive attributes that currently are being impressively tackled by modern models. And then that’s the whole question. What is really needed to make what is currently possible today effective and reliable? And then what is going to lead to developments that will help us rethink what’s possible with ecosystems of shared intelligence, which was how Friston et al. Put it in their 2022 work.
12:19
Speaker 1
Yeah, and it’s interesting that you say that because you can definitely see how this whole cycle of this loop, it increases the ability to make better predictions then, right? Because you’re refining your understanding and awareness of everything by taking things in through your senses and then acting on the environment to bring back more sensory input. That just helps you to gain more understanding, refine your perception, and then make better predictions. So when you’re talking about AI and you’re talking about what Versus AI. Is doing with the active inference, AI, their chief scientist, is, you know, it’s interesting because you can see how that would be highly important in a situation where, like you’re saying, you’re training these autonomous systems to be able to interpret the environment in real time.
13:17
Speaker 1
So it’s taking information through sensory awareness, whether it’s IoT, sensors or machines, devices, cameras, audio, all this different sensory input. Kind of like us as humans, we’re taking in our surroundings through our senses. And then with the Spatial Web Protocol, with the hyperspace modeling language programming context into the spatial awareness of all of the things in the spaces. So that also feeds in to this cycle to build awareness and understanding about the environments. So then you have the structure of all these individual intelligences that are learning off of each other and growing off of each other in that same cycle and feedback loop. So it’s like this whole collective intelligence that is all made up of all of these interactions that are performing the same refinement.
14:17
Speaker 1
So what are your thoughts on know you know, you’ve had me on your know, you’ve had Carl Friston on your show several times, right? A couple of times. And then Maxwell Ramstein, who is the director of research. Carl Friston is chief scientist. Maxwell is the director of research. You’ve had Mao and she’s one of the research scientists. So I know you’ve had a good glimpse into what Versus AI is doing using this methodology. What are your thoughts?
14:58
Speaker 2
Yeah, big questions. Of course, I only know from the open source education and research side. I can point to some of the reasons like why researchers in academia as well as those who are industry, those who are interested in applying active inference, they may turn to it for a variety of reasons and this doesn’t mean that it’s a rip and replace solution. Not everyone is using it in some first principles bottom up way. I think that’s super cool and exciting though, where it is possible, it increasingly is possible. But in a lot of settings, to my understanding, it’s part of a larger approach and so that’s something to keep in mind. People are always like blending the way that their systems currently work or their research questions currently work and then kind of bringing a yes and with active inference into the picture.
15:50
Speaker 2
So a few things though that I guess you could say patterns that we see across the hundreds of live streams that we’ve done and dozens of papers, colleagues from many different fields. A big feature that people point to is the interpretability or the explainability of the models. Because at the heart of an active inference generative model is an explicit graphical representation of what is being modeled rather than a massive kind of megaparameter uninterpretable instrument. There’s something that’s a lot more interpretable, at least in principle. Of course it’s always down to the specific generative model how interpretable. But a lot of really sophisticated cognitive phenomena can be modeled with very interpretable, explicit and legible models. That’s pretty cool across areas. Another feature that really excites people is just the action embeddedness.
16:50
Speaker 2
So if you just wanted to have something that’s like sitting and waiting then all you need is a passive model. But if you want to have behavior in the loop in this unfolding system, you need to really explicitly censor action. And that’s why there’s so many publications like, just to kind of give an example of this, predicting the price of stocks and cryptocurrencies and all these different kinds of commodities, and there’s academic papers that are publishing algorithms on it. And yet, if it was able to be used in a proactive way, then that kind of information would not being published through that venue. Which is kind of interesting to think about. Going forward is a really different question than simply making sense. They’re not unrelated questions.
17:37
Speaker 2
Making better sense of the past and the present may help with going forward but that’s not to say that every single update from the past and present does help. And so active inference is just like a wide open palette, gives a lot of expressivity to explore these phenomena. That doesn’t mean that a given active inference model is going to work in a given situation. But the hope and the excitement is that this expressivity helps us build generative models that do really well and interpretably and efficiently and reliably and so on in specific settings, and then that can be used in a really customized and effective yeah.
18:22
Speaker 1
So it’s interesting because from what I understand, the reason that Carl joined Versus was because he’s been developing the active inference and the free energy principle for what, the last ten years or so. And he saw the spatial web protocol and the way the HSML can take human language and program it into contextual elements in a spatial realm. And when he saw that, from my understanding, he was like, oh, well, that’s the world model that we need to actually be able to put this theory into reality. And so that, to me, it seems like, as far as AI is concerned, active inference alone isn’t it, the spatial web protocol alone, isn’t it, but when they come together, there’s the magical pieces of the puzzle that enable it to actually be able to be put into practice. So that’s pretty interesting to me.
19:34
Speaker 1
Can you explain because I know that a lot of my viewers also hear the term Markov blankets, and I know that’s an important piece to the functioning of active inference and the free energy principle and this action perception loop. Can you explain kind of in simple terms to the viewers what exactly that means and what purpose it has in the system?
20:02
Speaker 2
Yes. So first let’s get a little history. You mentioned about the timeline with Professor Carl Friston and collaborators developing these different concepts. So maybe that’s a little helpful before we dive into the Markov blanket and see where it comes into play. So the lab of Friston and a lot of the collaborations had to do with neuroimaging and that included a lot of the really rapid and early development around technologies like fMRI, functional MRI, so dynamic MRI, time series, EEG, so time series with a really different statistical and biological basis and these different kinds of neuroimaging modalities that were being brought together statistically. And a lot of those neuroimaging studies were on people who were just sense making with incoming stimuli. You could imagine that’s kind of like a passive neuroscience experiment, somebody’s in the kind of neuroimaging scanner.
21:03
Speaker 2
And then there is a lot of statistical deconvolution and analysis that goes into understanding how different individuals or different groups are associated with differences in brain response or different contexts are associated with differences in brain response. That was happening in the early 2000s. Carl was increasingly working and thinking from a physics of sentient systems perspective. It’s a whole story to go back into the exact literature and how things exactly unfolded. I’ll just kind of speak about it as we understand or think about it right now. But in the early 2000s, as the statistical package that was for neuroimaging, SPM was becoming one of the most cited citations and most utilized packages in the entire field of imaging neuroscience.
22:00
Speaker 2
Carl was working in these kind of fundamental questions of the physics of cognition in the early two thousand s and this concept of a variational principle that would integrate a lot of different other kinds of theories and equations in the computational neurosciences was kind of being developed as this theory branch that was elaborating and generalizing beyond practice, which was just about that neuroimaging and the kinds of modeling that was being done in those labs. And then through the heading into the early 2020s there was a lot of increased activity and there was also increased articulation between free energy principle as a principle, active inference as more of an implementational or procedural or tradition of modeling type approach. So that’s kind of where we stand. Of course there’s also more to say there, but just wanted to clarify that’s a little bit of the timeline.
23:03
Speaker 2
Also during the early 2000s there was a lot of advances in so called Bayesian causal modeling. And Bayesian causal modeling uses Bayesian statistics and it represents Bayesian statistical models as networks, which is to say with nodes and edges like a social network. And the nodes are variables, they could be fixed or they could be changing or learnt and then the edges are statistical relationships between the variables. And a Markov blanket is a situation where you pick a node of interest and then you pretty much blanket around that node of interest and you call all the states around that node of interest the blanket states for that node. So aspects of the world are not internal or external or blanket states in and of themselves. These are labels that are assigned to Bayesian graphs.
23:58
Speaker 2
So it’s kind of like we’re describing a map, not the territory. And then also depending on which node we select and call internal, that is what is going to decide what nodes are then for that internal state, its blanket states. And then one of the key innovations that was brought in by Friston and colleagues was to think about that Markov blanket, that set of insulating nodes, not just as kind of like a membership list, but to associate the inbound statistical dependencies with perception and the outbound statistical dependencies with action. So that kind of brought together Bayesian causal modeling with cybernetics and cognitive systems.
24:44
Speaker 1
Interesting. So that makes a lot of sense to me because from what I understand about how the active inference AI works within the spatial web, it’s nested ecosystems and it’s based on this holonic structure and it uses Markov blankets. So that makes a lot of sense to me when you’re thinking about another aspect of how these intelligent agents that are working with each other understand hierarchy and interrelationships between the agents and the data sharing that’s happening and the belief updating that is happening across systems. So that’s really interesting. So what excites you about the future? Knowing everything that’s happening within the sphere of active inference, within the sphere of AI and the potential it has with AI, what excites you about the future. Daniel.
25:53
Speaker 2
I’d actually love to hear your response. I guess it’s also distributed across so many other conversations. But I mean, what’s exciting to you or what area? And then that will give me a little time to think.
26:05
Speaker 1
Sure. Well, to mean what excites me is I see that we are on this precipice of a very big change in how we live and operate and interact with each other and with our surroundings and with data and just the way we kind of experience life as humans. I feel like it’s about to really change. I feel like we’re about to enter this kind of augmented world where AI becomes our personal assistant. AI becomes almost like this second brain that we get to bounce off of throughout our day and helps us to parse all of the increasing data points and the exponential growth of all of this technology and how we interact with it throughout our days.
26:59
Speaker 1
So it’s really interesting to me that’s why I’ve been doing what I’ve been doing is for education, because to me, it’s important for people to understand what’s coming and the transition, what it means to them and how they can prepare for it, so that it’s a smooth transition and they can benefit from it. A lot of people do a lot of fear mongering around AI. But I think that has a lot to do with these principles. The fear comes from the uncertainty. The fear comes from the not knowing. So I guess then what I’m trying to do is mitigate that so that they can be more certain about certain aspects of it and understand more so that they can see ways in which it can benefit them and how they can really things they can look forward to.
27:51
Speaker 1
So yeah, that’s kind of my perspective.
27:55
Speaker 2
Awesome. Yeah, there’s a lot there. I’ll just give a few things. You mentioned education. I think that’s also really exciting. So just kind of giving an institute scale response. I think it’s really exciting to utilize new techniques of learning and engagement to develop processes for having live streams, archiving and indexing the transcripts and translating them, providing subtitles. It’s just really exciting in education to see how a lot of the tools are getting to a place where they’re really applicable to support broad global online education, different languages, different familiarity with math and formalism. And it just feels like a really exciting time in education, especially if there can be more interest in the area.
28:50
Speaker 2
But as the active inference ecosystem starts to build and become what it is, being able to have new kinds of education and anticipate and build upon a lot of what we’ve learned in the past and how we want things to be going forward, that’s really exciting. Understanding of our self cognitive science is really meta in the case of human sociotechnical systems and in self reflection. And so that’s where there’s a lot of connections with metaphysics and with phenomenology, the kind of study of experience and a lot of cool work, of course, coming from Ramstead et al. With an inner screen in phenomenology. But suffice to say that’s part of a large body of work that deals with modeling different kinds of cognitive agents and then one cognitive agent of interested humans.
29:51
Speaker 2
So I think that really infinite frontier of self understanding which is being provoked and expanded with digital technologies. But I don’t think that’s so simple either as just the inevitable deployment of more and more surveillance capitalism, for example. So what could that be that would be different but also not necessarily simply evenly, more technological, a more active conception of different kinds of systems in the environment and the kinds of dynamic and vital interpretations of life and mind that can enable is really exciting. And there’s a lot of insight there from, again, people in the actin’ecosystem with philosophy and performance communication and backgrounds like that, where they bring so much depth to thinking about embodied, enacted and cultured systems and then to be even to meet part way.
30:54
Speaker 2
Speaking as a behavioral research scientist with the quantitative frameworks that can even try to be in the conversation I think is really exciting. And then last piece you mentioned uncertainty and Grappling and surfing uncertainty as a famous book in the area by Andy Clark is known. And the way that increased understanding of cognitive patterns, for example, through pattern languages and more cognitive science in diverse intelligences. Can a broader and also more fine grained understanding of cognitive patterns and dynamics help us bound and accept and certify uncertainty? Make better sense making? Make decisions that are more aligned with our preferences and our values? Use models that have explicit preferences, talk about those preferences with each other, have that conversation and bring everyone to the same table even if it takes a different amount of time for different conversations to pass around the table.
32:01
Speaker 2
But having one would be quite different.
32:04
Speaker 1
Yeah, very cool. So tell me a little bit about the Active Inference Symposium that’s coming up. I know. It’s called Enacting Ecosystems of Shared Intelligence. Why the name and what can people expect from that? I will be publishing this episode before that. So this is something that can bring awareness for people. I know it is coming right up though. What’s the date?
32:33
Speaker 2
Yes, it’s on August 22, 2023. So this is the third of a type of symposium that we’ve organized at the institute called the Applied Active Inference Symposium because even going back years, it was always one of the most pressing questions like wow, this is cool. And then how is it applied? What does this look like in practice? And so we’ve had this annual series to highlight what applying active inference in some area looks like at that time. So the first symposium was a Carl Friston kind of one man show. We asked many questions. It was very rich and Carl provided a lot of context great information. The second symposium was last year, 2022, and that was focused on robotics.
33:20
Speaker 2
So we heard from a lot of different researchers and developments in robotics and focused on what does active imprints look like in the robotics setting. And for this year were kind of thinking about different positionings and different topics and also kind of bewildered with the diversity of use cases that were seeing as were looking across all these different applications of Active. And it was actually during a scientific advisory board meeting and having Carl and other advisors there as were talking about the symposium and what would make sense. And somehow we brought up the designing ecosystems of intelligence work.
34:03
Speaker 1
Yes.
34:04
Speaker 2
We were thinking about the ecosystem perspective that we take at the institute, which is like, we produce open source products and provide services and the ecosystem has to really be enacted. People have to really be in the game. And that means all these different kinds of stakeholders and learners of all backgrounds and different kinds of organizations. And Carl basically suggested just taking it in the exact same direction with ecosystems and enacting it. So we thought about what it looked like to go from that designing phase and then to construct and to enact or really just to do not just in mind, but indeed. And it is going to be live streamed, following the live stream. It’ll be rewatchable and the transcript will be published and also used to train language models.
35:01
Speaker 2
So fret not if you’re watching after the fact, hopefully there’s later symposia as well. And yeah, there’s maybe 15 presenters and guests from all over the world. It’ll be in two intervals, there will be a closing roundtable and it’s going to be awesome to hear about a lot of the different applications of Active imperents. One other kind of cool piece, just to note it because it’s upcoming, is in the registration form. There’s some optional questions like what would be useful for you and what are you curious about? And useful and curious are basically analogies to pragmatic value and epistemic value, which are the two parts of expected free energy. And so it’s kind of like a little bit of applying active inference in how we ask and then we are passing those responses to those questions back to the presenters.
35:55
Speaker 2
So then they literally know what is valuable for people in terms of what they’re curious about and what they’d find useful. And I think that just speaks to what’s possible with online science and with participatory ecosystems and the kinds of feedback loops and distributed systems that we can be a part of and just how early it is for organizing and including people that way, but how exciting it is. So all those things are really cool.
36:26
Speaker 1
That’s awesome. Yeah. And for viewers who aren’t familiar with it, the. Paper that Daniel’s referring to here. The Designing Ecosystems of Shared Intelligence. That’s actually the versus White paper. And I will include the link to that in the show notes as well as links to the symposium and how you can register real quick before we tie up, because you just mentioned that last year’s symposium was on active inference’s role in robotics. So I know that’s something that our viewers would probably be really interested to hear just a little bit about. What was that about and what is the role of active inference in robotics currently? And I know it’s probably evolved so much just in this last year.
37:14
Speaker 2
Yeah. Again, I can only say from what I know in the education and kind of facilitation space, but robotics is on the ground, or if it’s not on the ground, it’s real. It is really where discussions about embodiment and implementation come into play and in the world dealing with physical embodiment, wheels slip and things happen and unknowns, and all these kinds of different realities and regularities in environments, even controlled environments. So it’s just a totally different setting than the sort of in silico. And so active inference for a lot of the reasons that we’ve been discussing earlier, ranging from interpretability to some of the computational benefits and just the explicit optimization of a generative model, the composability of those generative models, and so on has motivated a lot of researchers in robotics to develop generative models that support different kinds of robotic systems.
38:19
Speaker 2
I do not know how broadly these systems are used or where they have specific advantages relative to alternatives, but the fact that it is coming into play, funded work and real serious work is in the area is a good sign. And it’s just so cool that we can have an umbrella big enough to include the robotics conversation alongside all these other cool topics that we’re bringing up today.
38:48
Speaker 1
Yeah, well, it’s interesting because it does make sense in the sense that with robotics, like you were saying, these are agents that are dealing with the physical world, so they have to be able to make decisions based on the unexpected.
39:06
Speaker 2
Because.
39:11
Speaker 1
They can’t predict 100% accuracy on their environment when there’s so many variables in the environment. So that makes a lot of sense. Yeah. So, Daniel, thank you so much for being here with us today. It’s always a pleasure talking with you. I always get a different angle on my thought processes in this space, so I really appreciate you. I appreciate all of the great work that you’re doing. And you’re really building a community for all of the people involved in research and just learning about this space. So I definitely appreciate everything you’re doing. How can people reach out to you? How can they become involved in the institute?
39:57
Speaker 2
Well, thank you, Denise. It’s very kind. And it certainly is a whole team colony effort. People can head over to activeimprints.org and go to our website, always see updated information, people who are curious to take learning groups, there’s always courses. Some are more with synchronous activity, others are more at your own pace, different levels, different approaches. We hope to improve and diversify our offerings to just meet you wherever you, the listenerlearner are at. So I hope that whenever people listen to this, if they’re inspired to learn active inference, apply it, learn how to think within this framework, challenge it, improve it, do all kinds of learning and research and application. I hope it remains an open field for people and that we can continue to enact the kinds of ecosystems that we really want to see. Science and technical areas have wonderful and.
41:06
Speaker 1
I will put all of your links in the show notes and thank you again. And thank you everyone for tuning in. If you’d like to learn more about the spatial web and active Inference AI, feel free to visit my blog, deniseholt us you can visit aspatiawebfoundation.org they are the organization that is kind of working with the IEEE project where the core standards being developed around the spatial web protocols, the HSTP and HSML. And then you can also visit versus AI and find out a lot of resources around all of the technological breakthroughs that seem to be announced pretty much daily these days within this space, and just all the great work that they’re doing. So thank you again, Daniel, and thank you everyone, for tuning in and we’ll see you next time.
The convergence of two groundbreaking technologies is reshaping how we think about AI, automation, and intelligent systems, affecting every industry...
On 29 May 2025, the IEEE Standards Board cast the final vote that transformed P2874 into the official IEEE 2874–2025...
Explore the future of agent communication protocols like MCP, ACP, A2A, and ANP in the age of the Spatial Web...
Fusing neurons with silicon chips might sound like science fiction, but for Cortical Labs, it represents what's possible in AI...
Ten years ago, on March 31, 2015, I interviewed Katryna Dow, CEO and Founder of Meeco, to discuss an emerging...
We stand at a critical juncture in AI that few truly understand. LLMs can automate tasks but lack true agency....
VERSES AI 's New Genius™ Platform Delivers Far More Performance than Open AI's Most Advanced Model at a Fraction of...
Go behind the scenes of Genius —with probabilistic models, explainable and self-organizing AI, able to reason, plan and adapt under...
By understanding and adopting Active Inference AI, enterprises can overcome the limitations of deep learning models, unlocking smarter, more responsive,...